Recently, there has been an explosion of interest in AI across all industries. SMEs and enterprise organizations aim to ride the wave of AI excitement to expand their reach and discover new revenue opportunities.
Product companies are eager to tap into the power of machine learning—to do so, they are building and expanding AI engineering teams.
At the time of writing, the demand for AI engineers is growing by leaps and bounds. According to the US Bureau of Labor Statistics, the need for AI developers will increase by 23% over the next decade, outpacing most industries.
Staying up-to-date on AI engineers’ skills, average salaries, and responsibilities helps make intelligent hiring decisions in a cutthroat market. We created a typical AI developer CV based on our experience building AI/ML models and systems at Xenoss. Tech teams can use the AI engineer skills and responsibilities list for reference in hiring.
To avoid common pitfalls team leaders fall into when hiring AI engineers, look at our hands-on guide for hiring skilled professionals. If you are interested in tips for structuring your ML development process in the most resource-efficient way, head over to our guide on AI development.
AI engineer responsibilities
We broadly grouped responsibilities into technical and business categories to offer a high-level understanding of the AI engineering job function without focusing on specific technologies.
This distinction highlights that, aside from building functional tech projects, AI engineers are crucial in business consulting and strategic planning and help foster understanding between technical and non-technical stakeholders.
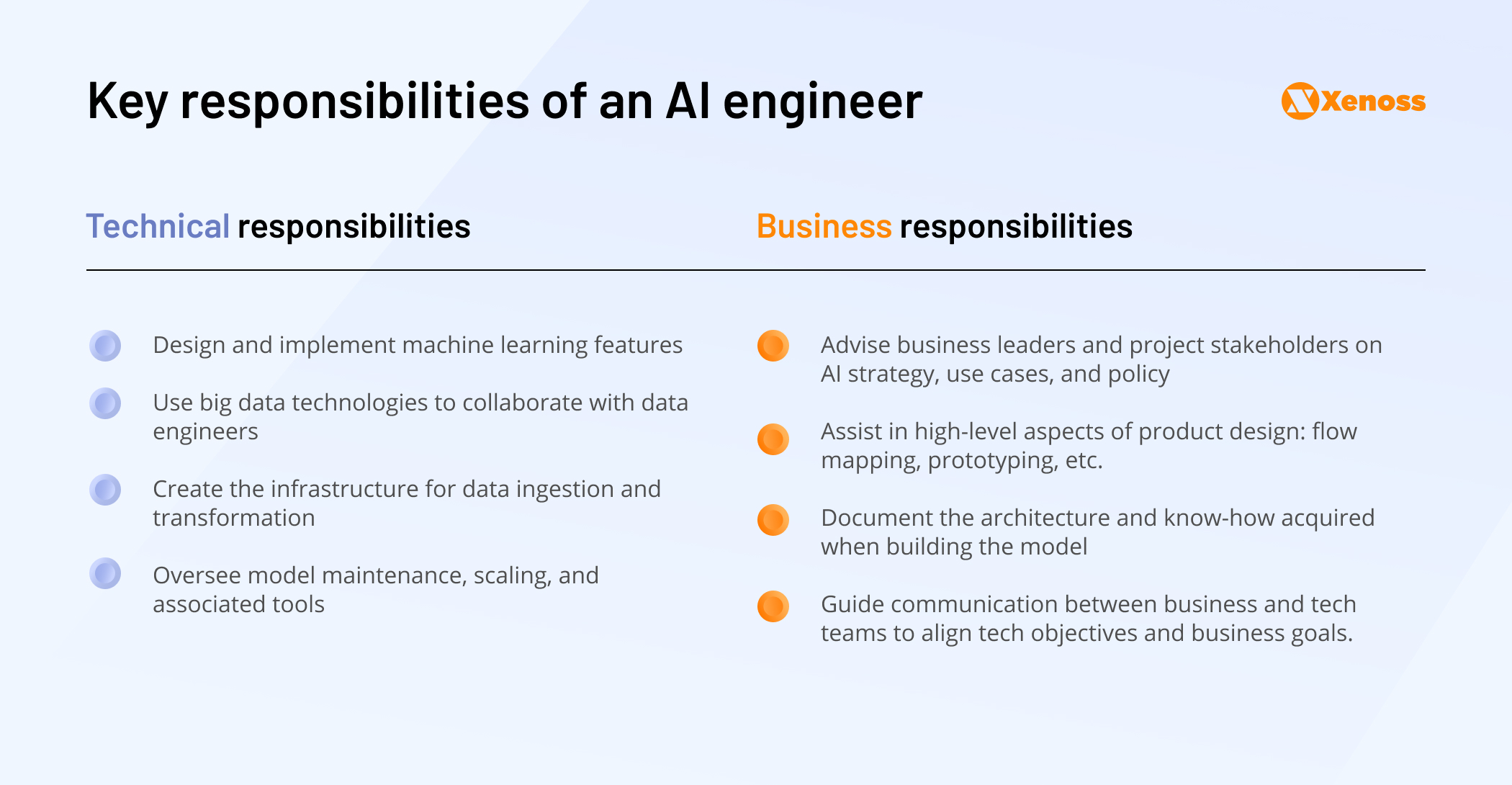
Data engineer vs. Data Scientist vs. AI software engineer
AI engineering, data science, and data engineering are intrinsically linked — AI (artificial intelligence) algorithms work with data collected and processed by data scientists. Likewise, data engineering helps lay the foundation for successful ML models through building scalable pipelines, data warehousing, and modeling.
Each of these roles is vital for a reliable AI-enabled product, but it’s essential to understand the function and responsibilities of these functions. This way, tech team leaders can identify the role that matches the needs of your project.
In the table below, we help draw the line between the responsibilities, skills, and prerequisites for hiring data engineers, data scientists, and AI engineers.
We would like to point out that there’s some discussion about the difference between ML and AI engineering roles. Some argue that ML engineers are responsible for designing models while AI engineers focus on deployment.
In practice, the terms “AI” and “machine learning” are used interchangeably by a large share of the AI (artificial intelligence) community, which will be our approach.
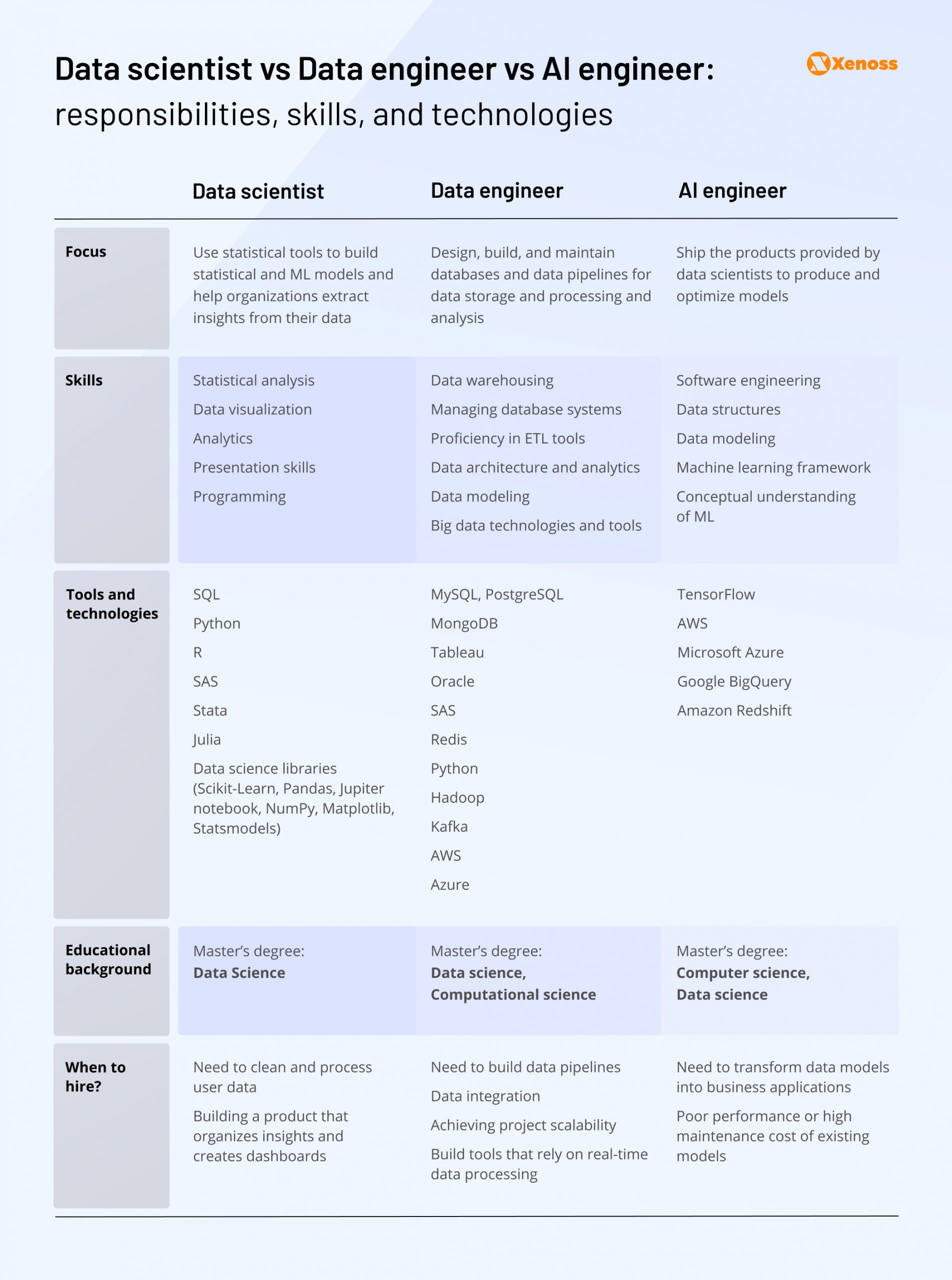
AI engineer skills to look for in job candidates
Tech team leaders should rely on something other than a cookie-cutter approach when hiring AI (artificial intelligence) engineers. Specific domain knowledge, the ability to match the product’s needs, and experience that matches particular tasks should be considered alongside machine learning skills.
Here’s a recap of crucial tech qualifications usually expected from someone on the AI software engineer path.
Technical skills
- 2+ years of experience in building AI models
- Proven track record of using Python, TensorFlow, and deep learning in business applications
- Experience with NoSQL, RDBMS, and REST API development
- Advanced mathematics (linear algebra, calculus) and algorithm knowledge
- Tech skills should be backed up by AI engineer certification (by IBM, Google, and others)
Non-technical skills
- Ability to interact with the product team and align business goals with technical outcomes
- Continuous learning and readiness to stay up-to-date with AI (artificial intelligence) development trends
- Creative thinking and problem-solving that enable AI engineers to find practical solutions to seemingly complex tasks
- Prioritization and time management: ability to work under pressure and tight deadlines
Domain expertise
- Ability to identify correct data sources for AI models
- Understanding of domain-specific metrics for evaluating the success of the use case
- Ability to use domain expertise for feature selection
- Readiness to keep track of domain-specific challenges and coming up with ways to solve them by applying AI tools
Xenoss AI engineers have a solid commitment to lifelong learning and following the trends of our key domains: MarTech and AdTech. Understanding the processes behind real-time bidding, contextual targeting, and other processes allows us to build machine-learning models that bring tangible value to the end user.
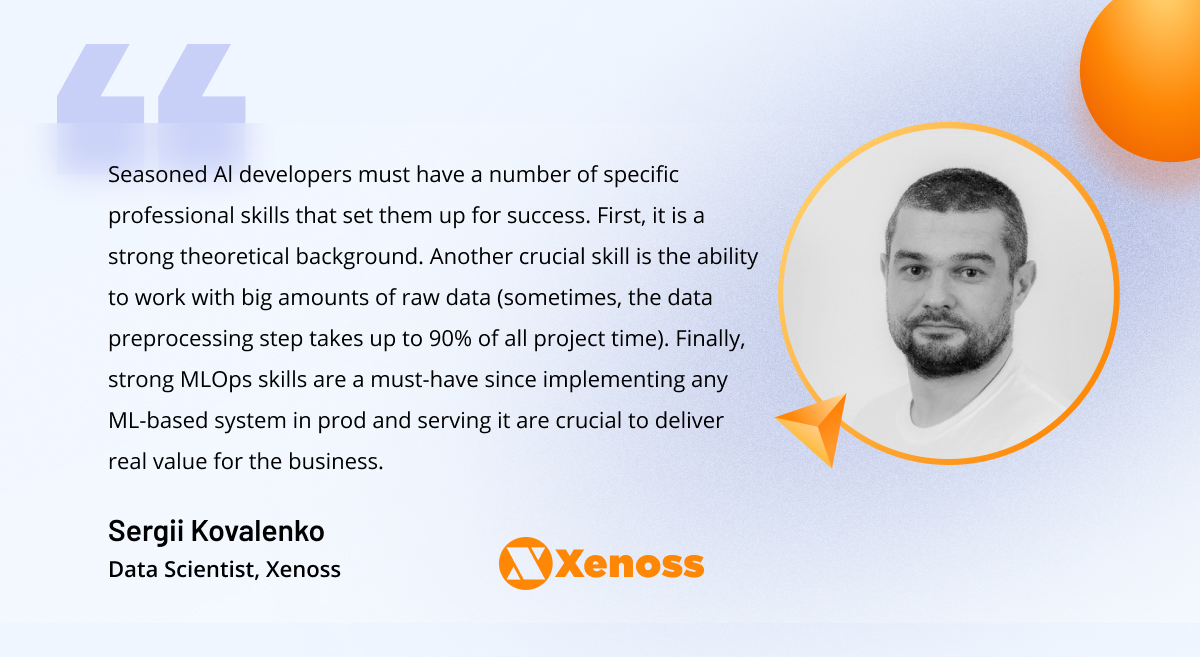
For example, Xenoss engineers understood the need to work around performance constraints typical of high-load systems when building an ML-based behavior prediction model for a mobile DSP. By choosing algorithms with minimal latency, our engineering team was able to leverage innovation without compromising performance.
In a different project, we found an innovative way to deploy computer vision for ad creative management.
In both cases, a deep understanding of the AdTech ecosystem helped build platforms with a tight product-market fit.
For a clearer understanding of the experience, skills, and education background to look for in AI engineers, take a look at our sample AI software engineer CV, crafted based on the expertise of Xenoss engineers.
How much does an AI engineer make
As Big Tech companies reignite their interest in AI products, more AI engineers are inclined to join these workplaces. To compete with the name recognition, job security, and benefits of working at FAANG, lesser-known companies try to attract talent by offering AI engineers higher salaries.
According to the US Bureau of Labor Statistics, the median software engineer salary is $136,600/yr. However, there’s a lot of variance depending on the location—Bay Area, Boston, or New York City engineers are, on average, paid higher than developers in other US states.
Similarly, when looking for AI research scientist talent, it’s worth exploring salaries outside America.
To give tech teams a broader outlook on AI/ML engineer salaries worldwide, we explored data by experience and location.
By experience (data by Glassdoor):
- Junior AI engineer: $124,000/yr
- Middle AI engineer: $138,000/yr
- Senior AI engineer: $151,000/yr
By location:
US:
- San Francisco Bay area: $138,000
- Bellevue, WA: $173,200
- Boston, MA: $166,500
- New York: $153,600
Europe:
- UK: £60,646/yr
- Germany: €71,400
- France: €58,100
- Italy: €36,600
Top certifications for AI engineers
While screening CVs and assessing the prior experience of AI (artificial intelligence) engineers at interviews is quite effective, it’s helpful to have other reliable selection criteria. Certifications released by AI industry leaders can confirm a hire’s deep learning, computer vision, model deployment, and optimization skills.
Here is the list of widely known and recognized AI certifications you can expect to see from skilled AI developers.
Intel Edge AI Certification
Intel Edge AI Certification is a way for engineers to prove their competence in building edge AI products.
Skills measured by the certification:
- Develop computer vision models
- Optimize models for performance on various hardware types
- Design deep learning applications
The Intel Edge AI ML certification requires engineers to use APIs and libraries for PyTorch, TensorFlow, and ONMX.
Microsoft Certified: Azure AI Engineer Associate
The certification confirms the engineer’s ability to design, build, and deploy machine learning models using Azure AI. Certified engineers are expected to contribute to every model design and testing phase.
Skills measured by the certification:
- Building a design for an AzureAI solution
- Implementing computer vision tools
- Applying NLP
- Using document intelligence and knowledge mining tools
- Implementing generative AI solutions
IBM Machine Learning Professional Certificate
The credential helps confirm an engineer’s skills in Deep Learning, Reinforcement Learning, and Supervised Learning. It also tests AI developers on Survival Analysis and Time Series Analysis.
Skills measured by the certification:
- Exploratory data analysis
- Unsupervised learning
- Supervised learning: classification and regression
- Deep learning and reinforcement learning
- Specialized models: time series and survival analysis
Google AI engineer certification
After completing the courses on machine learning offered by Google, AI engineers can showcase their skills through the certification in a Professional Machine Learning Engineer exam.
Skills measured by the certification:
- Architecting low-code machine learning models
- Developed prototypes into functional algorithms
- Scale machine learning models
- Orchestrate and automate pipelines
- Monitor AI solutions
Final thoughts
As AI becomes ubiquitous, users and investors alike expect product companies to have AI tools in their products. Tech teams are scrambling for skilled and affordable engineers to design, ship, and maintain these features.
Hiring an in-house AI (artificial intelligence) engineering team is time-consuming and cost-intensive, especially in highly competitive locations. To reduce talent costs and time-to-market, tech companies are exploring alternatives like outstaffing.
Having a small core team of in-house engineers and a wider team of outstaffing experts with robust expertise and domain knowledge is becoming a widely accepted model.
Xenoss, a reliable outstaffing partner with over 15 years of experience, has a strong AI/ML engineering pool. Our developers helped many product teams strengthen their offerings with deep learning, computer vision, natural language processing, and other technologies.
Get a free consultation with our team to learn how Xenoss can help you build an AI engineering team to transform your product with accurate and valuable ML models.