Getting your Trinity Audio player ready... |
Despite being around for a few decades now, Artificial Intelligence (AI) still manages to rouse excitement at its mere mention. According to a PwC report, AI could add as much as $15.7 trillion to the global economy by 2030. According to the Artificial Intelligence Index Report by Stanford University, the global investment in marketing AI at the start of 2023 reached over $3 billion.
AI is a game changer for many industries, including digital marketing. After all, there is a constant stream of user data that can be used to deliver marketing insights. AI has found a comfortable place within marketing technology and is used by marketers to provide better personalization, perform competitor analysis, predict customer behavior and much more.
In this article, we will take a look at various ways AI has been transforming marketing technology and what the future holds for it.
What marketers expect of AI the most
According to a recent study by Narrative Science, 38% of marketers agree that the most important benefit AI can provide is an accurate prediction of consumer behavior.
Furthermore, 27% of marketing specialists cite manual and routine processes automation, and 14% point to the AI’s ability to provide real-time monitoring and alerts, among the most important benefits of AI implementation.
AI and machine learning applications in marketing
We’ve divided the main uses of AI in marketing by technologies behind it and their respective applications within the industry to get the full picture of the AI transformation.
Seeing as the marketing industry has only just begun to dip its toes into intelligent technologies, we will also look into the future AI applications.
This will allow us to understand where MarTech is heading, what to prepare for, and what to consider before embedding AI into custom MarTech software development.
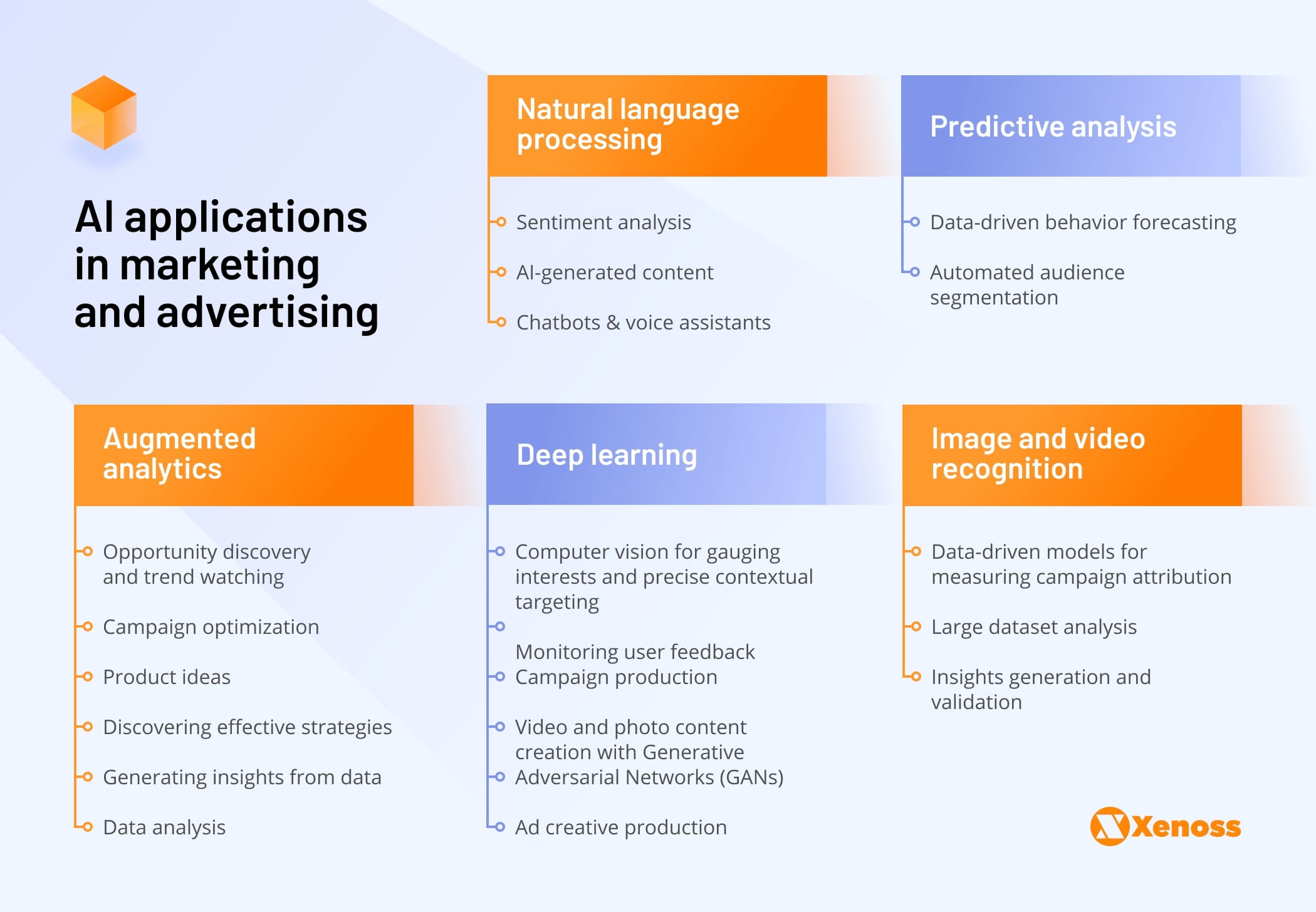
Natural Language Processing
Natural Language Processing (NLP) aims to teach computers to understand and derive meaning from human languages. This is done by analyzing large amounts of natural language data. Over the years, NLP has seen increasing uses, including chatbots, search engines, social media tools, etc.
Let’s take a look at how NLP works within marketing technology.
Sentiment analysis
Sentiment analysis, also referred to as opinion mining, has many applications for marketing. Sentiment analyzers process text or speech and can evaluate the context, emotion, and intent behind it.
Currently, there are already tools on the market (like a readily-available pre-trained algorithm called VADER) that utilize sentiment analysis to help companies perform brand monitoring, analyze the market and competitor products, gain insights from customer reviews, and much more.
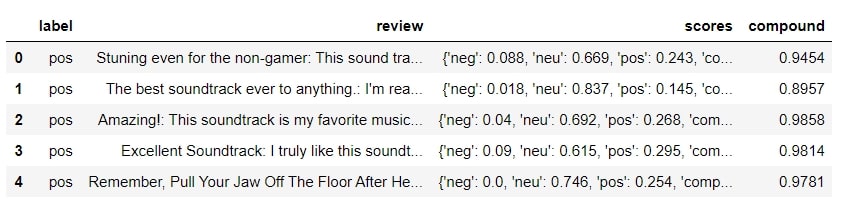
For example, there are tools like Lexalytics that analyze what customers say about a company and explain why they feel a certain way about a product.
Sentiment analysis is a constantly evolving field. Ultimately, such tools will go beyond identifying what is said and determine the tone behind it, such as sarcasm or irony.
This will further help companies understand their customer data better and drive marketing efforts in the right direction.
Streamlining content creation
Content marketing remains one of the most effective tools in digital marketing, but it’s a lengthy and expensive process. In times when we are constantly bombarded with information, making content that stands out becomes increasingly difficult. With the help of NLP, AI tools can identify interesting topics, generate actual content and personalize it.
One of the best examples of what AI is capable of when it comes to content creation is presented by ChatGPT, which can now run content that matches the brand’s tone of voice and the expectations of a specific target audience.
Conversational AI and voice AI
By releasing ChatGPT and introducing an accessible GPT API, OpenAI started a chatbot revolution, putting thousands of chatbot applications on the market. Many marketing chatbots are helping automate all facets of marketing operations: fetching keyword reports and SEO data.
There’s also growing awareness of the opportunities voice AI brings to the table. 88% of companies surveyed by Deepgram are already using voice technology, and 66% see it as an important tool for their organization’s future plans.
When it comes to conversational intelligence, tools like ChatGPT have their gaps and shortcomings. However, the spike in AI research and investment (in 2022, VCs poured $4.5 billion into AI projects) will likely help large language models quickly mature and become more reliable in customer support and experience.
GPT is not the only contender in the LLM space: both Google and Meta introduced their own models – PaLM2 and LLaMA, respectively.
Predictive analytics for behavior forecasting
With the marketing industry shifting from traditional methods to digital, the amount of data available to MarTech companies has increased. Data is the new oil as long as that data is used correctly.
Thus, it makes sense to tap into predictive analytics, one of the most advanced AI technologies and one that has already been conquering the marketing industry.
Predictive analytics utilizes machine learning models to identify patterns in datasets and predict the future.
This type of analytics has found many applications within marketing. Forecasting customer behavior to deliver personalized experiences is perhaps the most widespread use of predictive marketing.
The way it typically works is as follows: AI algorithms first segment customers into different groups based on various factors either by demographics or how often someone visits a website, then a predictive model analyzes different segment groups and provides recommendations based on the historical data available.
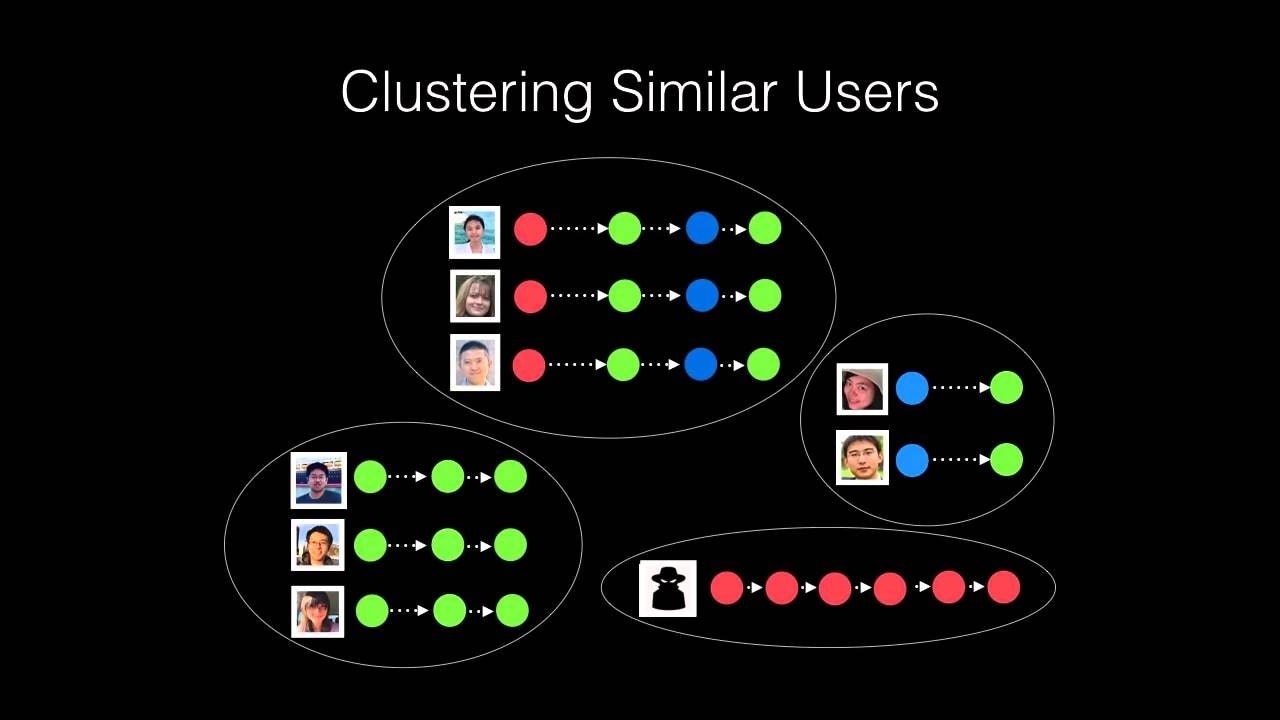
An example of an advanced AI-based predictive analytics tool that sets the benchmark for a new generation of analytics is Qlik Sense. This data analytics platform offers a set of APIs, scalable multi-cloud architecture, and an associative analytics engine with sophisticated AI.
The Associative Engine is an algorithm that helps you find relevant data without having to wade through irrelevant information. It makes it easier to sift through your data faster than ever before.
With intuitive data prep, drag-and-drop and drill-down features, you can quickly and easily spot and share data relationships and key insights.
Augmented analytics
While predictive analytics uses a lot of data to predict what will happen in the future, augmented analytics uses context and knowledge to flag insights to alert marketers to be more effective.
Augmented analytics is an intelligent approach to leveraging cognitive computing to extract more meaningful insights from your data.
This AI-based approach allows brands to intelligently augment their data-driven processes to discover new patterns, relationships, and trends they might otherwise miss.
When we take into account user intent and behaviors, the insights generated are context-aware and highly relevant. Based on the contextual nature of the insights, marketers can generate human-centered product ideas based on an in-depth understanding of their user needs.
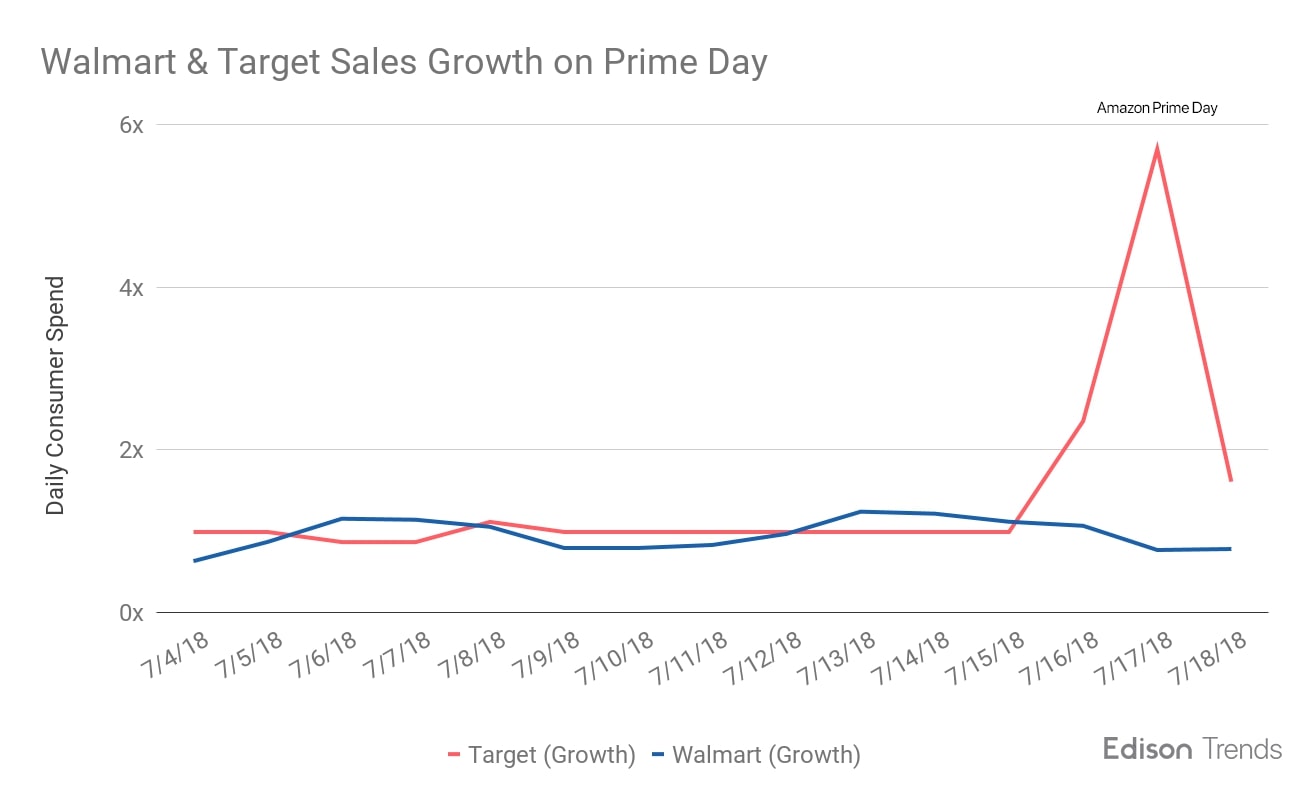
Augmented Analytics algorithms detect schemas, profile, catalog, etc. and recommend enrichment, data lineage, and metadata.
By analyzing natural language queries, algorithms find all relevant patterns in data and enable the auto-generation of models. Then insights are narrated in natural language or visualizations with a focus on what is important and actionable.
These models can be embedded in mobile apps and conversational AI to yield the best results.
One of the leading augmented analytics platforms is Sisense Sense-IQ. It provides AI throughout the platform to help anyone make sense of data by automatically highlighting impacts and insights. Anyone can type a question to get forward-looking insights and enable deeper investigation of data regardless of their skill set.
Image and video recognition for contextual advertising and campaign production
Contextual targeting
Amid the decline of cookies, contextual targeting has been at the forefront of possible solutions as a less privacy-invading option. But the question of how to reach the same levels of accuracy with contextual ads as with cookie-powered advertising remains.
While an NLP-based solution can analyze the textual content of the page and display ads based on what’s being talked about, text is only oftentimes just half the content on a webpage. What about the rest, such as images?
There are a couple of MarTech solutions available, such as GumGum, that harness the power of computer vision to “see” what’s in the images and derive context from it.
Then using that data combined with the context from the text, they display the most relevant ad to the user. So for example, it would be a good place to advertise winter, next to a picture of a car in the snow.
Gathering user feedback
Image recognition is extremely helpful in tracking campaign performance.
A growing number of adtech platforms add eye-tracking tools to help advertisers discover how users interact with ads.
Processing this data can give marketers a clear view of which branded elements appeal to target audiences and help design creatives that resonate with prospects.
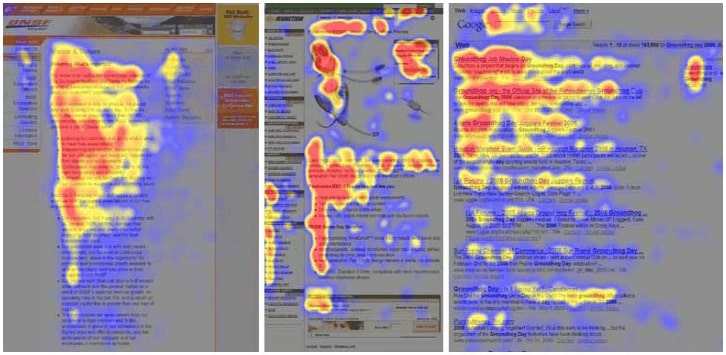
Content generation
According to a survey by Digiday, 71% of marketers at creative agencies found ways to use generative AI in their work. Out of these, 75% rely on ChatGPT and the like to write copy and 73% use it for research.
Among brands, content creation is the most common use of generative AI. Retail is also one of the fields likely to be heavily impacted by large language models: 96% of retail executives acknowledge the game-changing potential of these technologies. The role of artificial intelligence will be seen both on a customer’s end (in AI-driven experiences like those created by Macy’s or Starbucks) and in facilitating the way teams process disparate insights, transforming them into actionable strategies.
Google also rolled out an AI-enabled offering for retail. Product Studio provides a suite of tools designed to assist small businesses in enhancing their product images (adding custom product scenes, eliminating blurry backgrounds, and improving image resolution). The tool will help retailers streamline the process of listing products in the Merchant Centre.
In the last fifteen years, we’ve helped our customers strengthen their retail technology offerings with AI in different ways: from ML-empowered analytics to marketing automation and ad performance management platforms.
It is interesting to see how the growth of AI fits into the broader narrative of other tech megatrends: 5G, cloud computing, first-party data infrastructure, and others. To be ahead of the curve of change, we are surveying industry leaders on their predictions for the future of advertising and marketing technology. To share your experience and insights, take the survey.
AI in multi-channel attribution
The number of marketing channels keeps growing, and as advertisers try to expand their reach, there’s a need for a more accurate way to measure omnichannel campaign attribution.
Traditionally, marketing attribution would use heuristic methods to assign touchpoints to conversion paths. It could either be last-click, first-click, linear or customized. However, all these models oversimplify consumer journey, introduce bias, and are overall less accurate.
Data-driven attribution models, also called machine learning attribution models, use a company’s own historical data to assign credit to different touchpoints instead of arbitrary rules.
One challenge associated with data-driven attribution is that there is no way to test them before deployment. You can’t compare the model’s output with some source of truth data.
That being said, data science and AI are constantly evolving, and in the grand scheme of things, AI is still at its inception stage. Data-centric companies are coming up with ways to solve this issue.
Dataiku, for example, simulates user journey and then picks one channel that will result in a 100% conversion rate to see if the model will be able to assign 100 credit to the channel.
As more research into machine learning becomes available attribution models can be expected to become more accurate. There has recently been published a paper that proposes the use of a deep neural network called DeepMTA for better interpretability.
It takes into account three important factors in the customer journey: event sequence order, event frequency and time-decay effect of the event. When evaluated on a real dataset, the model was able to achieve 91% accuracy.
How to approach AI implementation into MarTech solution
When you don’t need AI
It’s very tempting to want to include AI in your product, after all, it’s all the rage nowadays. However, AI for the sake of AI has very little value and meaning. AI implementation is often an expensive and resource-intensive venture.
AI, and specifically machine learning methods are used to infer predictions and discover patterns from existing data. Oftentimes, when companies think they need complex ML models to solve a problem, it can be solved with more traditional approaches.
Businesses don’t often understand that to train AI you need large amounts of quality data.
On top of that, you need the infrastructure to support the processing and analysis of said data. Before you even consider AI, you first need to analyze the trade-offs between time and money invested and the value you will gain.
A new study by Forrester suggests that although 88% of businesses are already using AI, the vast majority – 55% – are dissatisfied.
In part, it’s due to the fact that they started investing in AI without having properly assessed their needs, data availability, and overall readiness to jump on the AI bandwagon.
The more data you can feed to your AI solution, the more accurately it can simulate human thinking and learning. AI is a great solution to automate non-repetitive processes and tasks that involve natural speech understanding or image recognition.
The rest of the time, traditional business process automation (BPA) and statistics would do.
When you do need AI
You’ve weighed all the pros and cons but still aren’t sure if AI is for you. How do you know if you have a good use case for AI within your product? Thankfully, there are some clear signals that can help. For example, if your product handles large amounts of data or your work processes involve a lot of manual tasks this signals a potential AI use case. Any manual task automation could have AI potential within it.
Not sure? Bring experts in.
Find a company that has a successful track record of developing AI solutions. Tech partners can help you identify features and tasks within your product that can be improved with AI. On top of that, they will also be able to tell if your product is ready to have AI implemented.
So let’s sum up.
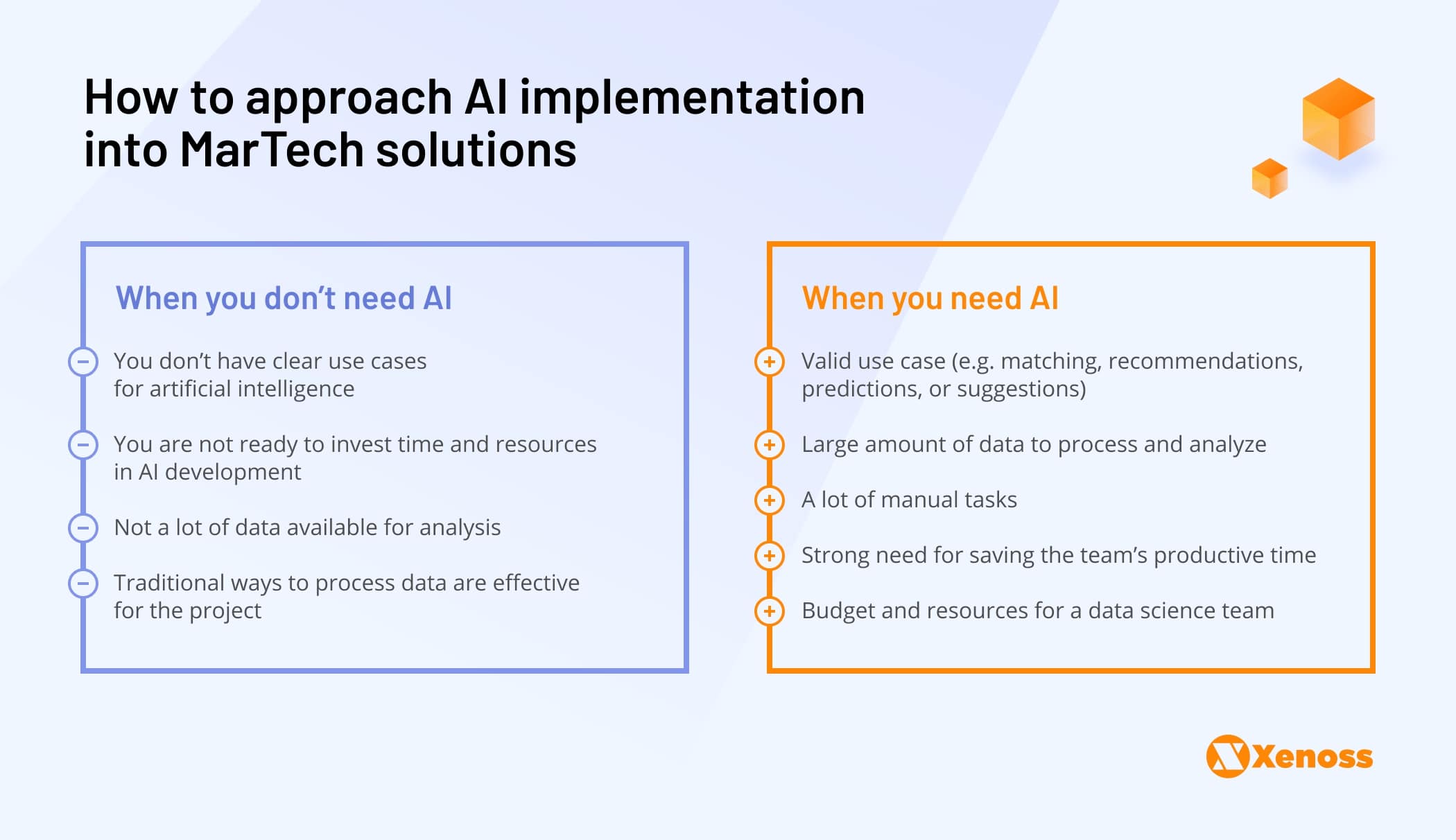
Conclusion
AI is continuously bringing innovation to the marketing industry across all segments. It’s able to effectively address many challenges the industry faces.
Companies looking to improve their product with AI need to validate their value proposition and ensure tech maturity before committing to the project.