If you are a non-technical professional working for an organization that is building machine learning models (either internal or user-facing), you may have encountered machine learning engineers discussing model entropy during technical talks.
Although entropy is a technical term, understanding it is also essential for non-technical professionals (business leaders and product owners) working with AI/ML, as it is the concept behind accurate predictions, more innovative model design, and a reduced risk of poor decisions.
In this post, we offer a comprehensive definition of entropy in machine learning and a rundown of its business impact. Followed by part 2, on how entropy is used in decision trees, and part 3, where we compare entropy with the Gini index.
You will learn:
- What entropy is and how it is connected to the concepts of “surprise” and information gain
- Why non-technical leaders benefit from understanding the concept of entropy
- What the most common use cases of entropy are in machine learning projects.
What is entropy in machine learning?
A layman’s understanding of thermodynamics is usually sufficient to remember that entropy is a measure of disorder in a given system.
When Claude Shannon designed information theory in the 1940s, he adopted this concept previously used in physics to describe the degree of surprise in the system.
Shannon’s definition directly links an increase in entropy to a gain in information. When someone says something others already know (low surprise), it means no new information was communicated.
However, if someone shares an unknown and unexpected detail (high surprise), new information is learned from the message.
For a practical understanding of what “surprise” is, let’s use an analogy commonly deployed by machine learning engineers: Coin tossing.
A real-world example of entropy in a dataset: A coin toss experiment
When a coin is flipped, it typically shows up heads (H) or tails (T), allowing us to assume a 50% probability (p = 0.5 in technical terms) of either event.
Suppose an improbable event occurs (e.g., instead of showing heads or tails, the coin shows something else). In that case, the person recording the outcomes will likely be reasonably surprised, indicating an increase of entropy in the system.
Hence, the relationship between “probability” and “surprise” emerges.
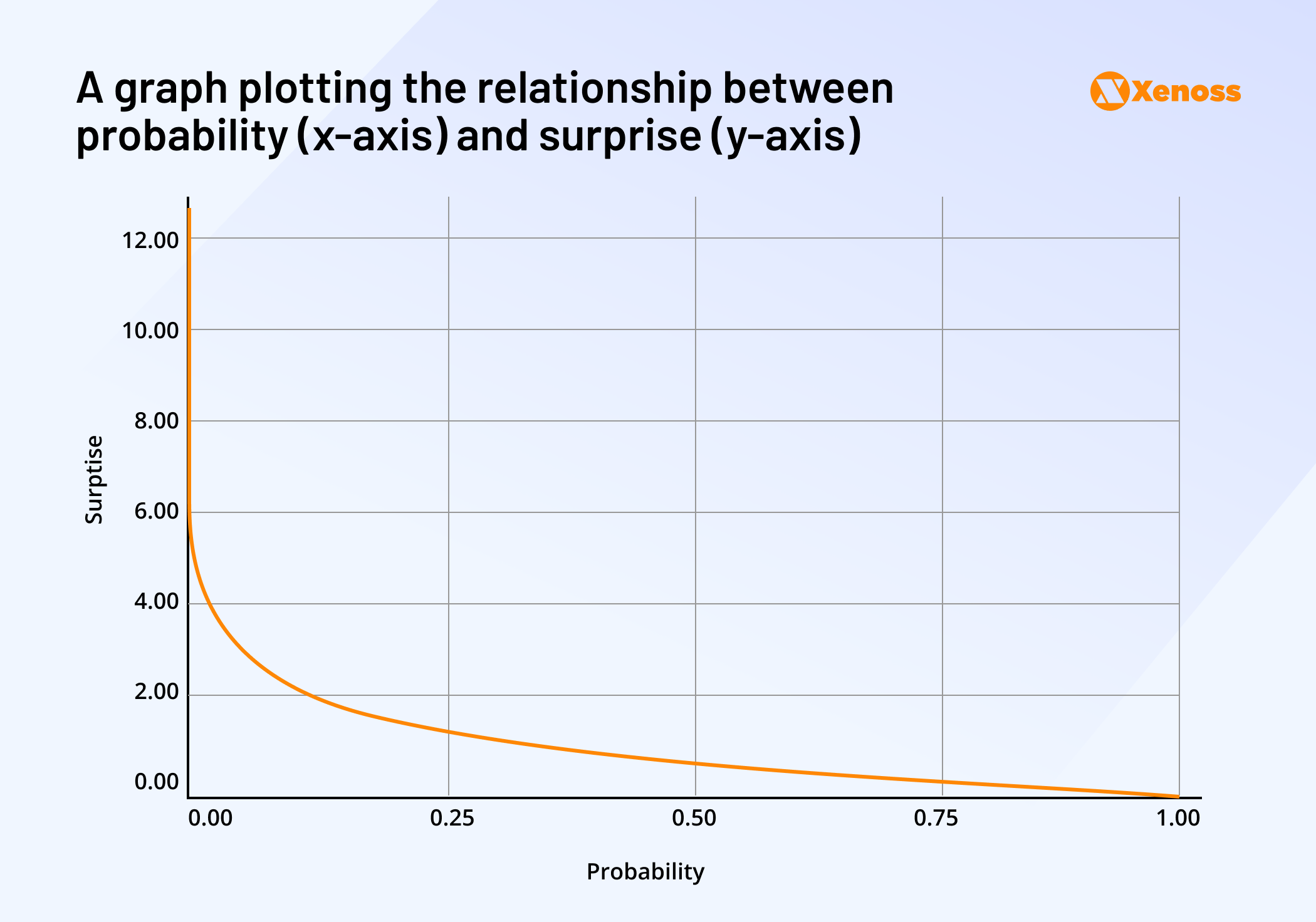
Understanding the graph:
- As the probability increases, the degree of “surprise” is reduced, reaching zero when p = 1.00 (an event always has the same outcome). Remember that events with p=1.00 are usually theoretical and do not exist in reality.
- Smaller probability values, on the other hand, generate higher surprise levels.
In the context of surprise, entropy is the average of expected surprises for all possible outcomes linked to a random variable in machine learning.
A standard entropy graph for a probability distribution would look like this.
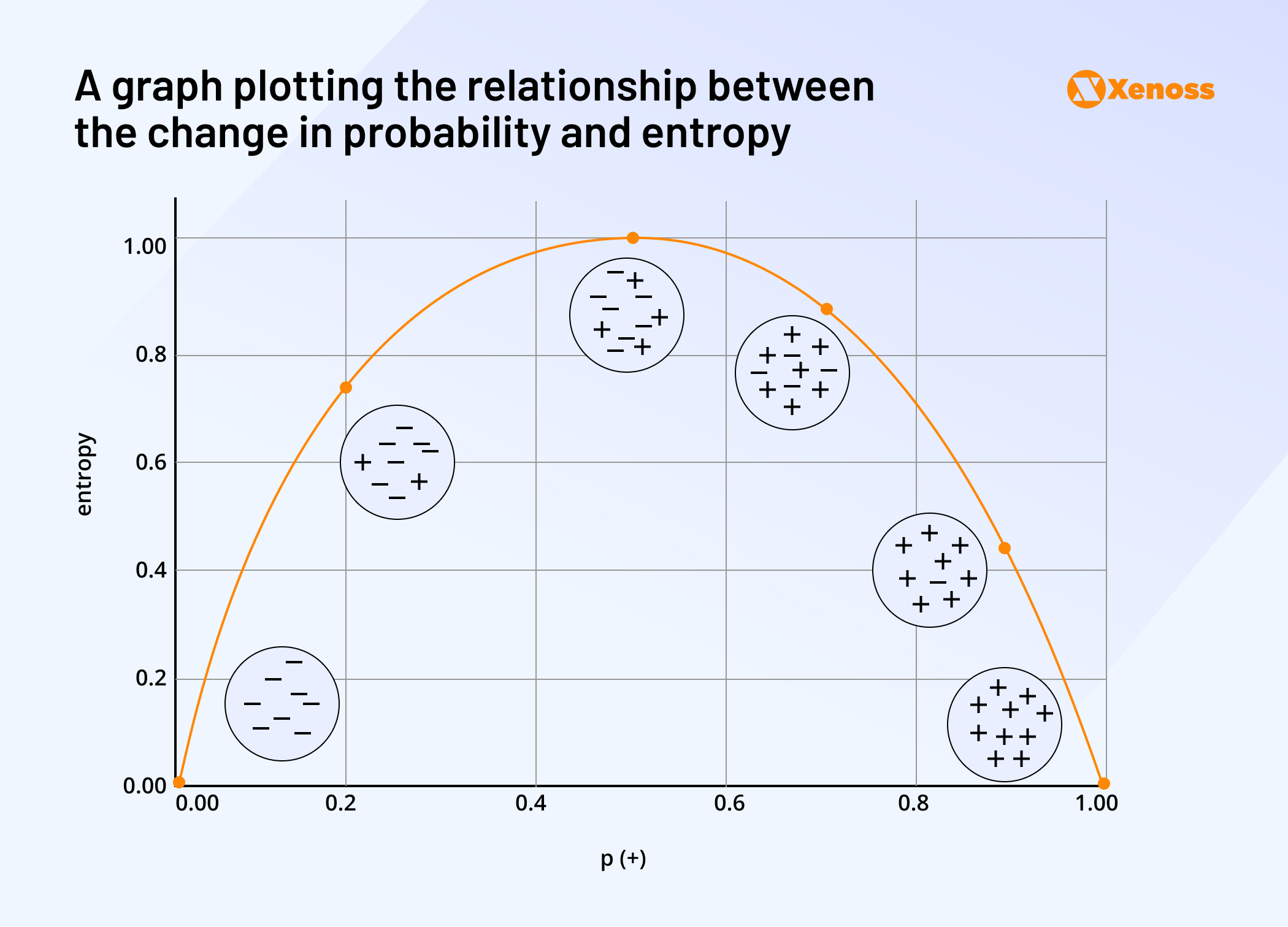
Note that entropy peaks when there’s an equal likelihood of multiple events (p = 0.5) and tapers off at the extremes of probability values.
In part two of the series on entropy in machine learning, we will discuss how entropy is applied to decision trees and examine different types of data entropy.
Business impact of entropy
The concept of entropy in machine learning is relevant for non-technical leaders as it offers deeper insight into three critical areas of model management: Prediction accuracy, feature selection, and reduced risk of overfitting.
High entropy impacts prediction accuracy
- Entropy indicates uncertainty within a dataset
- High entropy suggests the model may be unreliable
- High entropy reflects the need for more data or refined model training
Why improving prediction accuracy drives business value: The higher the accuracy of machine learning predictions, the better businesses can allocate resources (e.g., marketing spend or inventory) to reduce wasted investments and maximize profitability.
More precise predictions also improve customer experiences by delivering relevant services or products, strengthening loyalty, and driving extra revenue.
Calculating entropy facilitates feature selection
- Entropy is used to compute information gain, a metric that calculates how much new information a feature adds to the prediction.
- Calculating both the entropy and information gain associated with a feature allows machine learning engineers to choose the features that yield the most accurate outcomes.
How improving feature selection drives business value:
By selecting only the most impactful variables for a prediction task, businesses streamline model complexity and reduce computational costs and noise.
Improved accuracy and performance of machine learning models helps both improve internal decision-making and build more effective user-facing features (e.g., designing advertising creative assets with the highest probability of conversion).
Tracking entropy helps prevent overfitting
- Keeping track of entropy helps reduce the complexity of the model
- Eliminating less reliable features prevents the algorithm from being too specific for the training dataset.
How preventing overfitting drives business value: By ensuring that models generalize well rather than simply memorize training data, businesses get consistent and reliable insights that hold up in real-world scenarios.
The ability of machine learning applications to accommodate change determines the quality of decision-making and a stronger return on data-driven investments.
Bottom line
In the first part of the series on entropy in machine learning, we focused on the definition of entropy and its impact on the business value of machine learning models. Essentially, the higher the entropy in a machine learning model, the more uncertain predictions become. That’s why machine learning engineers select low-entropy features.
In Part 2 of our series on machine learning entropy, we examine how entropy helps ML teams streamline decision trees. In Part 3, we discuss other concepts associated with entropy in data science and its computationally efficient alternative: the Gini index.