The F-score, or F1-score, is a metric used to evaluate the performance of a classification model, particularly in imbalanced datasets. It is the harmonic mean of precision and recall, providing a single score that balances both metrics.
Formula:
F-score interpretation:
- A high F1-score indicates both high precision and high recall, meaning the model is good at identifying positive cases while minimizing false positives and false negatives.
- The F1-score ranges from 0 to 1, where 1 is the best score (perfect classification) and 0 is the worst.
Variants:
- Fβ-score: A generalization of the F1-score that allows weighting precision and recall differently using a parameter β\betaβ, where:
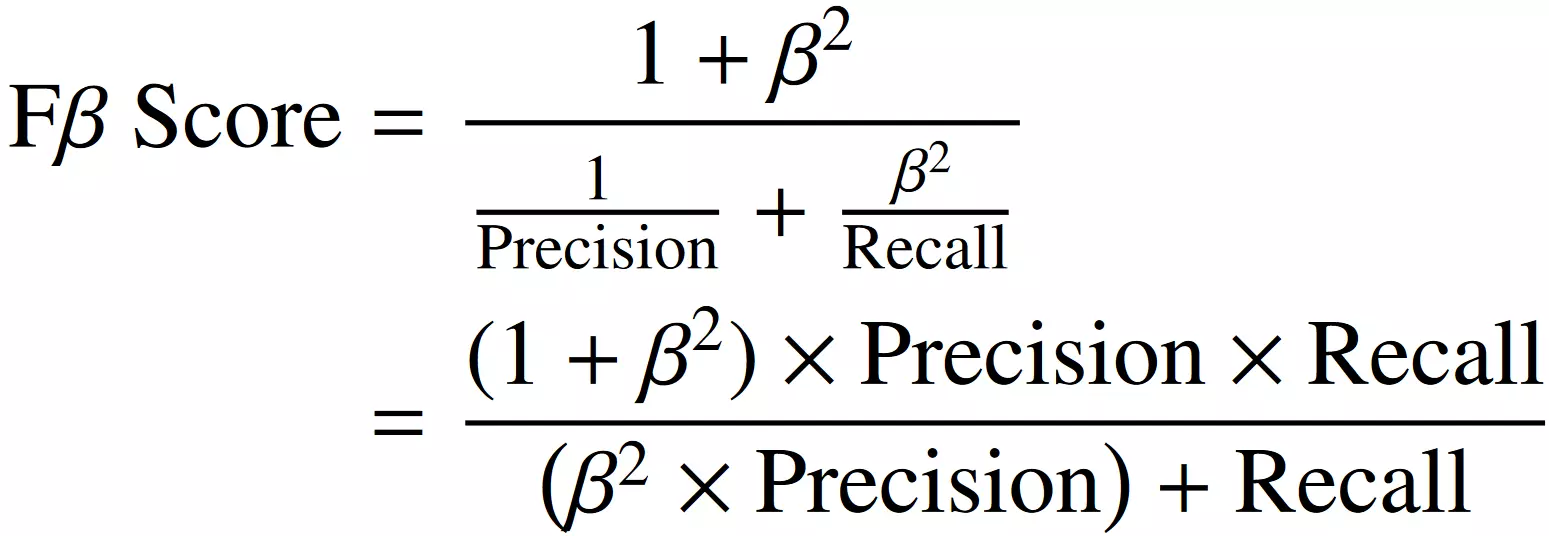
- If β>1, recall is weighted more heavily.
- If β<1, precision is emphasized.
- F2-score: Weighs recall higher, useful in cases like medical diagnosis where false negatives are costly.
- F0.5-score: Weighs precision higher, useful in cases like spam detection where false positives are more problematic.
Advantages and Disadvantages
Advantages
- Balances precision and recall: useful when one metric alone is not sufficient.
- More informative than accuracy: especially in imbalanced datasets where accuracy can be misleading.
- Applicable across domains: used in healthcare, cybersecurity, fraud detection, and NLP.
Disadvantages
- Does not distinguish between Type I and Type II errors: It treats false positives and false negatives equally, which might not always be ideal.
- Ignores true negatives: Unlike metrics like Matthews Correlation Coefficient (MCC), the F1-score does not take true negatives into account.
- Not suitable for ranking models: Two models with different precision-recall trade-offs might have the same F1-score, making comparison difficult.
F-score use cases
The F1-score is widely used in applications where precision and recall need to be carefully balanced, including:
Medical diagnosis
- Used to evaluate tests for diseases where missing a positive case (false negative) can have severe consequences (e.g., cancer screening).
- Preferred metric: F2-score, since recall is more important.
Spam detection
- Email filters use F1-score to balance precision (avoiding false positives) and recall (capturing all spam messages).
- Preferred metric: F0.5-score, since precision is more critical (avoiding false positives).
Fraud detection
- Banks and financial institutions use F1-score to detect fraudulent transactions.
- Preferred metric: F1-score (balanced approach) or F2-score if catching fraud is more important than avoiding false positives.
NLP & sentiment analysis
F1-score is used to evaluate classifiers for tasks like spam filtering, sentiment classification, and named entity recognition.
Information retrieval and search engines
Search engines optimize for F1-score to ensure relevant documents are retrieved while minimizing irrelevant ones.
Conclusion
The F1-score is a crucial metric for evaluating classification models, particularly when dealing with imbalanced datasets. While it provides a balanced measure of precision and recall, it should be used alongside other metrics such as accuracy, AUC-ROC, or MCC, depending on the problem’s requirements.
Choosing the right variant (F1, F2, or F0.5) depends on whether precision or recall is more important for the given application.