Getting your Trinity Audio player ready... |
Banking is both a promising and a challenging sector for AI adoption.
On one hand, the potential for automating repetitive finance operations is high: They are not inherently creative and would benefit from eliminating human error.
Analysts estimate that AI adoption can add up to $170 billion to the US banking sector by 2028. For major banks, AI excellence is becoming a crucial strategic objective; as Paul J. Davies put it for Bloomberg, “Every bank fears its competitors getting good at AI before they do.”
On the other hand, finance is a heavily regulated domain, and global government agencies are cautious about “unleashing” autonomous systems without human guidance and approval.
In this landscape, financial organization leaders need to understand which automation goals are too ambitious (e.g., a fully automated AI bank), which lack disruptive potential (disparate AI applications in banking like chatbots that are not embedded into the customer journey), and which offer the most transformative power without the risk of losing compliance.
This article examines four high-impact areas where AI in banking is already driving results:
- Customer service
- Cybersecurity, fraud detection, and prevention
- Loan underwriting
- Operational efficiency and automation
#1: Customer service
Amazon has raised the bar for flexibility, speed, transparency, and seamless delivery beyond e-commerce.
In banking, convenience and personalization are no longer differentiators—they’re requirements. McKinsey data shows that banks that prioritize customer satisfaction see faster deposit growth.
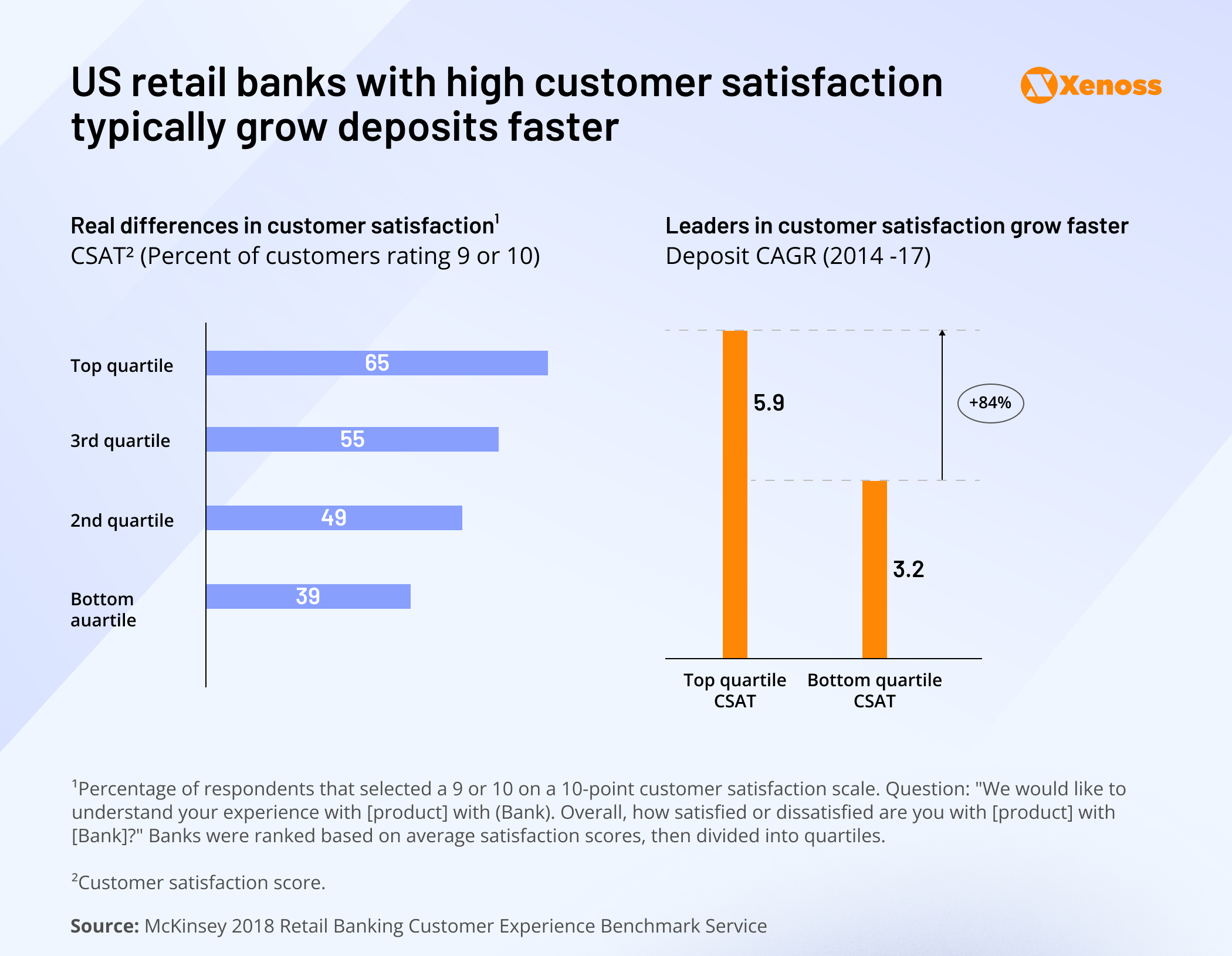
Additionally, banks are currently under the looming threat of disintermediation. The services that financial institutions alone used to handle (payment, budgeting, even loan underwriting) are now chipped at by non-banking innovators.
In China, Tencent-owned WeChat offers users a wide range of financial products. Google also has a budgeting and financial management tools suite. FinTech startups like Cropin use AI and advanced analytics to simplify the underwriting process.
In a landscape where financial institutions no longer monopolize their core capabilities, improving the customer experience with innovative technologies can help maintain the status quo.
How AI is changing the face of customer engagement in banking
An AI-first customer engagement strategy involves constant aggregation and analysis of user data, including needs, on-site behavior, context (such as type of employment, sources of income, family situation, etc.), and preferences.
Machine learning models offer a deeper understanding of these signals compared to traditional statistics-based algorithms like logistic regression.
Financial institutions can channel newly discovered insights into three high-impact areas.
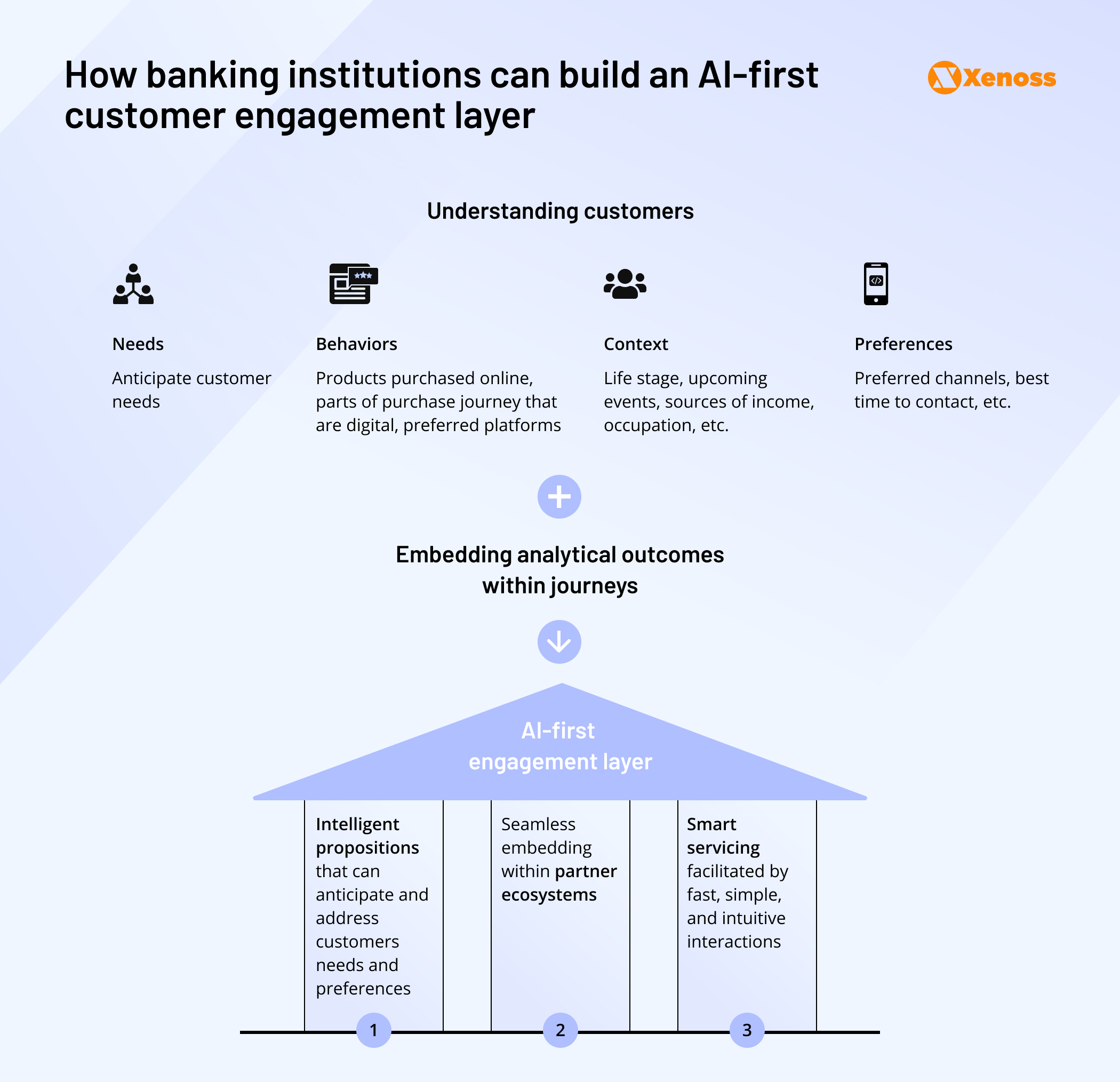
Intelligent product suggestions
Companies outside of finance, like Netflix, were able to attract and continuously expand their customer base with intelligent content recommendation engines.
Retailers also use AI-enabled product recommendations engines to recommend products that meet their needs.
For financial institutions, intelligent recommendation engines can also make a significant difference.
Personetics, an AI-driven personalization provider for banks, allows banks to embed product recommendations into customer journeys based on user behavior signals. These hyper-personalized suggestions helped financial institutions like Synovus, the 8th-largest bank headquartered in the US, improve their deposit growth rate and achieve a 19% conversion rate.
Cross-channel experiences across partner ecosystems
Financial institutions can counter the disintermediation threat by collaborating with partner ecosystems and leveraging the data these platforms collect to engage customers through the bank’s services.
Citibank was among the eight companies Google partnered with in 2021 to offer digital savings capabilities to Google Pay users. The deal gave the tech giant the infrastructure for building a fintech product, while Citibank acquired a new way to reach digital-only customers.
In October 2024, Citibank expanded its partnership with Google by leveraging its technology for expanding its use cases for artificial intelligence in banking.
Hyper-personalized wealth management tools
Machine learning helps banks use customer data to build services that keep customers engaged beyond financial operations, like wealth and liquidity management platforms or budgeting tools.
For instance, these real-world machine learning applications help improve customer engagement:
- The Royal Bank of Canada deployed an AI-enabled NOMI Forecast, a platform that offers realistic projections of future cash flows based on historical data. Over 900,000 bank clients have used this feature since 2021.
- Empower is a mobile app that helps reduce recurring expenses, such as subscriptions to rarely used tools or competitive mobile phone fees.
- Federal Bank built a virtual assistant that uses natural language and learns over time, improving the precision of its responses.
#2: Loan underwriting
Using machine learning models to accurately predict the probability of a lender defaulting on a loan is gaining traction among banks. Underwriters are using artificial intelligence to better use the data they have at their disposal, eliminate bias from decision-making, and get more insight on millions of citizens (45 million Americans, according to the Office of Research report) who don’t have sufficient credit data in their reports.
How the future of AI in banking impacts credit underwriting
Improving the accuracy of creditworthiness
Traditionally, banks use statistical tools like FICO (Fair Isaac Corporation) for default prediction and credit scoring. These systems consider a lender’s credit history, debt levels, payment history, and other variables to calculate default probabilities.
Though FICO-like models are still a gold standard in credit scoring, they are static and overly reliant on historical data, which is why these techniques don’t respond well to changes in a lender’s behavior or U-turns of the economy. Besides, traditional tools often overlook underrepresented lender segments, such as younger borrowers or those in emerging markets.
Financial institutions are aware of the reactive nature of statistical creditworthiness assessment, so they are turning to artificial intelligence.
Machine models help integrate data from disparate sources into a unified view and make a highly accurate default probability prediction.
Case study: Improving risk scoring with multimodal AI
Xenoss engineers supported a U.S. bank expanding into the Indian market in improving the accuracy of its credit scoring model using machine learning
Before reaching out to Xenoss, the client used independent statistical models to predict default probability based on the following data sources:
- Credit card transactions
- Account transactions
- Credit history
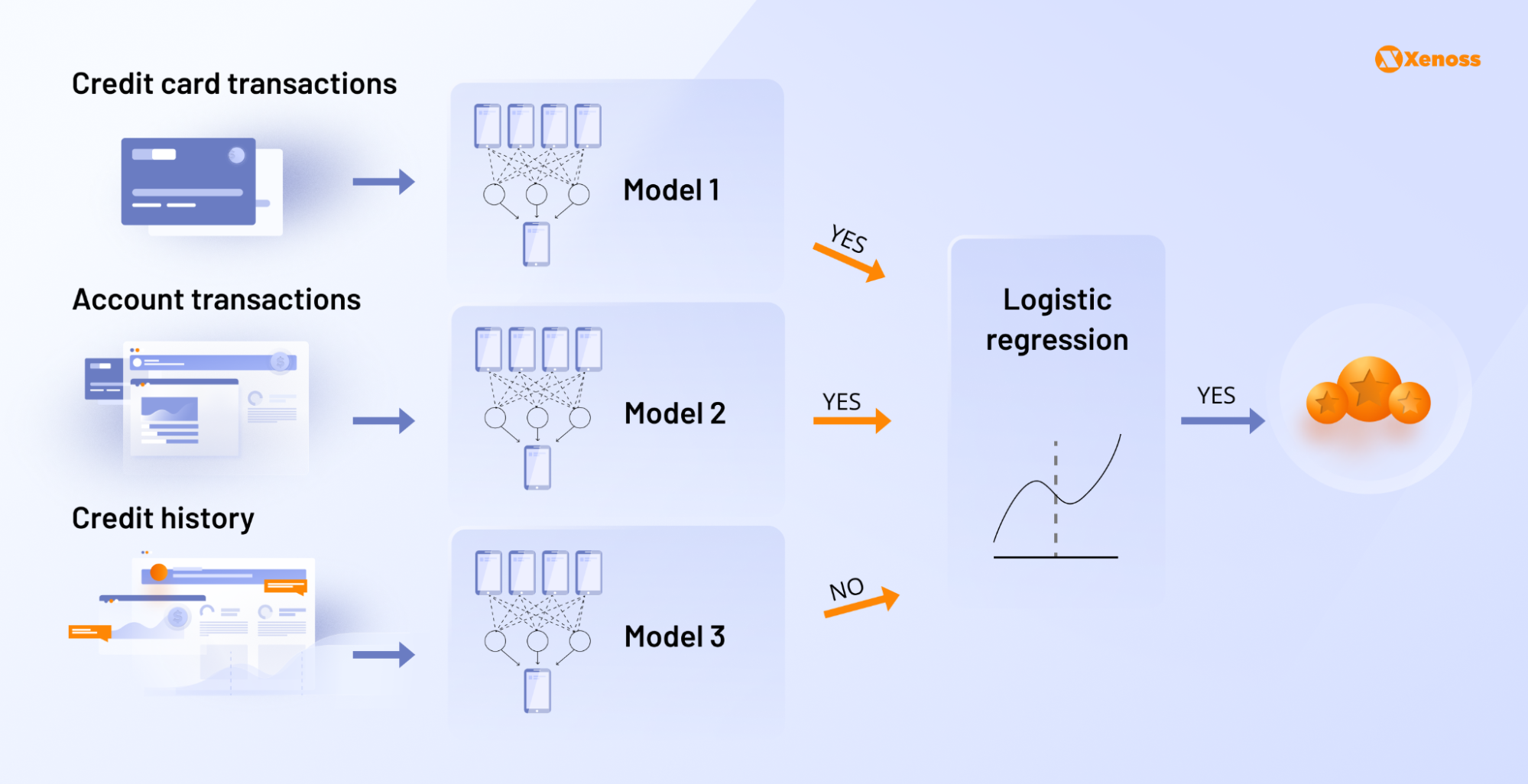
However, prediction accuracy was not high enough to support confident lending decisions, especially in a market with limited historical credit data. Seeking a more robust approach, the bank turned to Xenoss for technical guidance.
The machine learning engineers built a unified multi-modal neural network with embedded input to improve prediction accuracy.
In the new model, inputs from three data sources were transformed and fed into the unified neural network, which processed all the data at once and provided a 360-degree view of a customer profile based on a combination of credit card transactions, credit history, and account data.
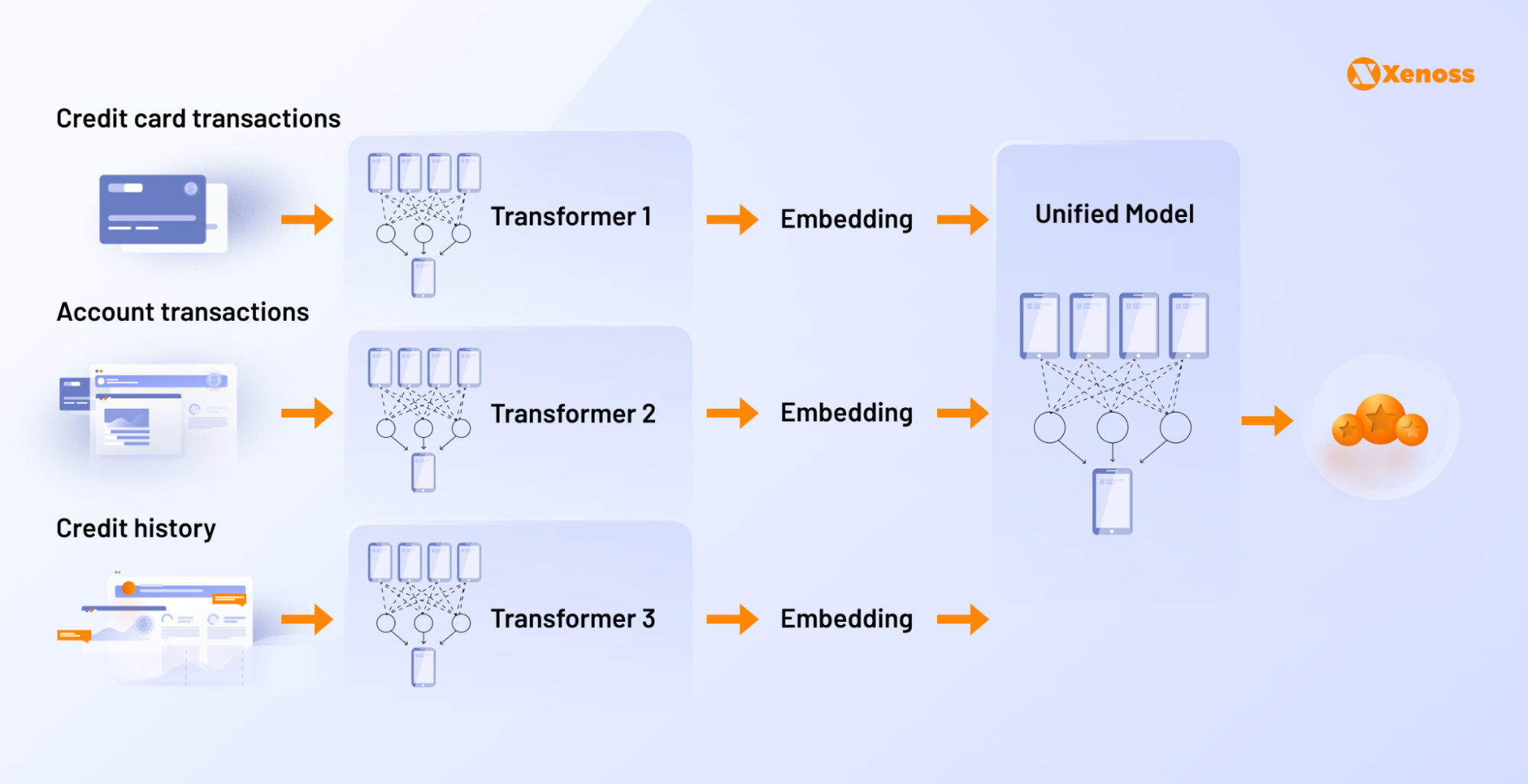
The shift to a new neural network yielded a 1.8-point Gini uplift—a metric that assesses the predictive accuracy of the model—and allowed the bank to precisely estimate the risk of default without needing to add new data sources.
#3: Cybersecurity, fraud detection, and prevention
Fraud takes many forms: identity theft, insider fraud, partner deception, customer scams, and payment tampering. This puts a significant strain on the global economy. According to Nasdaq, eliminating fraud could add 0.4% to the US GDP.
How AI improves fraud detection and prevention in banking
AI capabilities can help security teams detect much more granular signals of suspicious intentions than a human brain is capable of picking up.
Here are several effective real-world use case examples of machine learning applications for successful fraud detection and prevention.
- Image analytics. Ping An, a Chinese financial services holding company, designed a proprietary computer vision model to analyze 54 involuntary microexpressions that occur before a person can control their facial expression, to spot high-risk customers.
- Assessing cybersecurity risk in real-time. Generative AI in banking helps organizations write code for fraud detection rules, streamline “red testing” (simulating attack scenarios to choose the most effective response), or aggregate historical data from security events to pinpoint trends and root causes of vulnerabilities.
- Fighting financial crime. Banks increasingly use genAI to flag suspicious activity in real-time, based on a customer’s transaction history, and create customer risk ratings based on changes in know-your-customer (KYC) attributes. Oracle, for one, is pioneering the use of AI agents in countering financial crime. Oracle’s Investigation Hub enables autonomous workflows that assist investigators in collecting evidence, prioritizing actions, and documenting cases—automating manual steps without removing human oversight.
#4: Internal operations automation
In a volatile economy, banks focus AI efforts on proven use cases that reduce costs and boost operational efficiency. Rather than betting on experimental ideas, many are turning to ML to streamline everyday processes with minimal investment and fast time-to-value.
Here are the critical areas financial institutions should explore to “do more with less” in day-to-day operations.
Empowering the workforce
Augmenting employee productivity with machine learning is one of the safest yet impactful machine learning applications in banking.
Automating manual tasks like data entry and analysis, process documentation, and summarizing large amounts of corporate data can save teams across virtually every department of a large bank hours of productive time.
Compliance teams, in particular, have benefited from using genAI tools to summarize and extract key takeaways from the latest regulations.
Transforming technologies
As large-language models become increasingly on par with humans in programming, banking organizations can use these tools to optimize existing code, reducing the time in-house teams need to build proprietary technology.
A Portugal-based financial organization built an internal banking AI engineering copilot that migrated the company’s core technology from outdated COBOL-based platforms to Oracle. The tool not only handled the code migration but also generated documentation and metadata schemas to support long-term maintainability.
How to foster successful AI adoption
Xenoss’ engineers noticed that three success determinants help companies achieve maximum returns from AI adoption with minimal risk.
Build a centralized data hub
Data is the foundation of every AI initiative. Banks need well-governed, real-time access to structured and unstructured data across departments. This requires a strong data governance framework, secure storage, and minimal latency between data generation and consumption.
Optimize every layer of the tech infrastructure
Scaling machine learning is nearly impossible on top of outdated systems. Legacy infrastructure creates bottlenecks that limit performance, agility, and integration.
That’s why reviewing and modernizing foundational technologies should come before deploying AI applications. A strong, flexible core is what allows AI to scale efficiently and deliver sustained value.
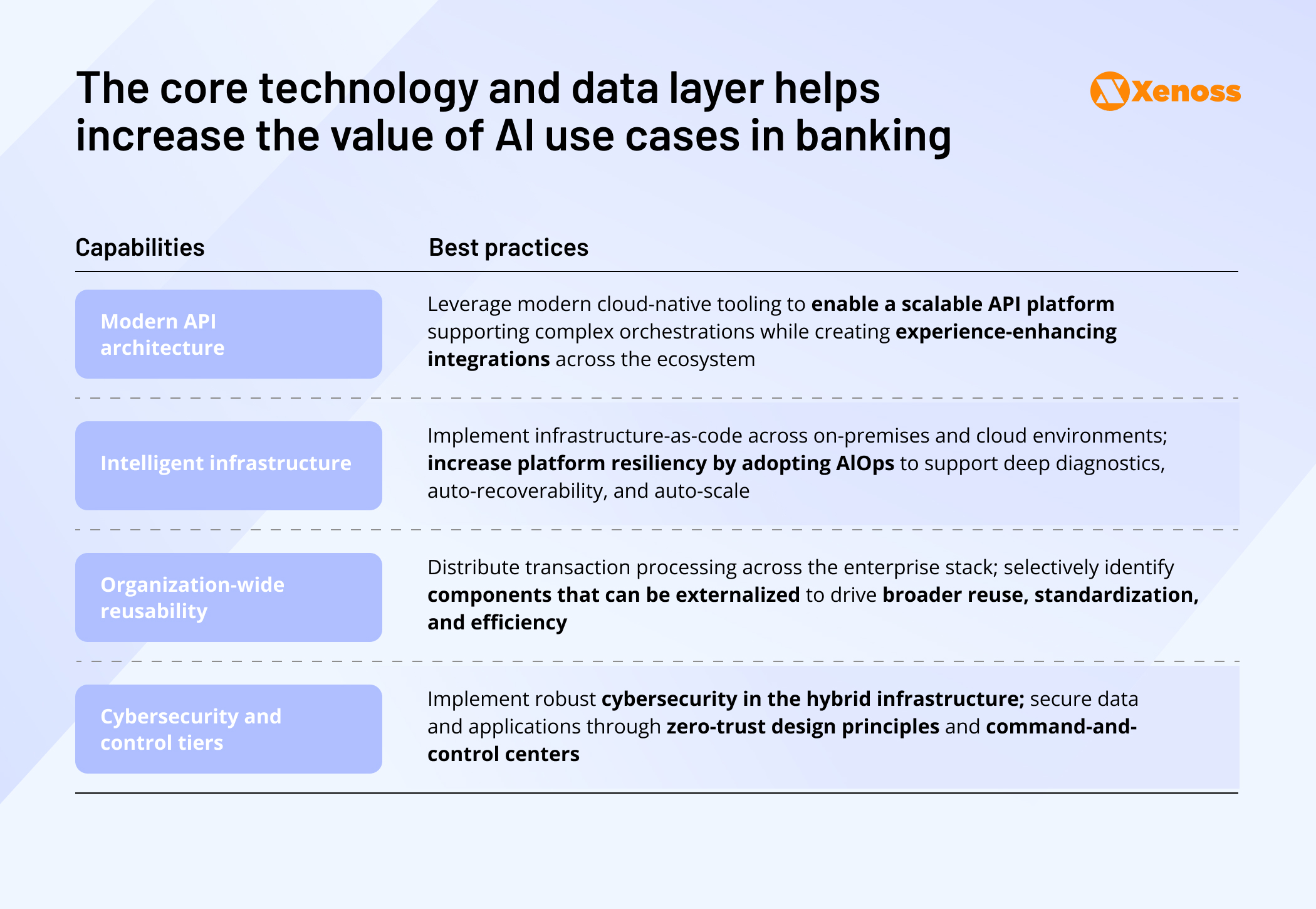
The following components are the building blocks of rolling out AI for financial services.
- APIs strategy. Connecting internal systems via APIs reduces silos, improves data exchange, and enables integration with external partners—unlocking broader AI use cases in banking.
- Infrastructure-as-code. A hybrid architecture with on-premise control and cloud scalability reduces maintenance overhead and accelerates deployment.
- Codebase standardization. Ensuring that the bulk of AI components are reusable across the banking infrastructure promotes standardization and reduces time to market.
- Security and governance: Adopting zero-trust principles and implementing centralized control centers ensures compliance and strengthens risk management.
Commit to organization-wide transformation
In Xenoss’ experience, banks that succeed with AI are those willing to implement it across entire business domains, not just in isolated, point-based use cases. Broad adoption leads to greater impact and operational consistency.
Crucially, this kind of transformation depends on well-oiled MLOps processes—repeatable, procedural, and decoupled from day-to-day operations, allowing banks to scale AI in banking and finance without disrupting core workflows.
Bottom line
As AI and banking continue to evolve and digital startups raise the bar for customer experience, traditional banks are starting to rethink how they connect with their customers.
The most forward-thinking institutions aren’t just adopting AI to optimize processes—they’re embedding it at the core of their strategy. From personalization and fraud detection to infrastructure and underwriting, AI is becoming a catalyst for reimagining the entire banking value chain.
Getting there, though, isn’t just about plugging in new tech. It demands bold leadership, new talent, deep partnerships, and a commitment to modernizing core systems.
Banks that treat AI not as an add-on, but as a driver of transformation, will shape the future of finance.