Getting your Trinity Audio player ready... |
On February 2nd, OpenAI released Deep Research, a powerful research agent tool that responds to user queries with in-depth analysis and detailed reports.
Deep Research offers a more autonomous approach to analyzing sources and synthesizing data than run-of-the-mill LLMs. It can download and read PDF files, write scripts, synthesize graphs, create tables, and alter its reasoning depending on the data it finds online.
The release led to a massive hype wave, with leaders eager to find high-yield use cases and white-collar workers worried about job security. Yet, even though the technology under the hood is impressive, beneath the buzz lies a strategic question: Can tools like Deep Research truly drive strategic insight and deliver actionable value?
To find out, the Xenoss team ran a focused test on one of the most promising Deep Research use cases: market research. A human team selected a complex and data-rich industry—bioinformatics—to evaluate how well it gathers data relevant to a company’s area of interest (machine learning and data engineering).
What makes tools like Deep Research a breakthrough compared to traditional LLMs?
Deep Research stands out from traditional LLMs—including models like GPT o1—by combining advanced reasoning with autonomous task execution.
To understand how OpenAI’s Deep Research operates, let’s dive deeper into how it approaches reasoning and agency.
Reasoning
With advanced reasoning models like o1, OpenAI shifted from non-chain-of-thought to chain-of-thought models.
Non-chain-of-thought models create answers word-for-word by reproducing the likeliest pattern of joining words with one another. They did not reason but regurgitated millions of documents they had been trained on. This limits LLMs’ ability to deliver structured, innovative, or inferential responses.
Switching to chain-of-thought models drastically improved LLM performance, especially in reasoning-heavy areas like math and logic.
Deep Research leverages CoT models (GPT o1 and o3) to deliver more structured and inferential output.
Agency
What separates Deep Research outputs from the standard web version of ChatGPT is its ability to act autonomously: Navigate different browser tabs, download files, read code, and create visuals.
While OpenAI has a general-purpose agent, Operator, Deep Research is research-specific. It uses GPT o3 and agentic powers for complex research workflows, producing structured outputs like graduate-level reports in a sleek workflow, as shown below.
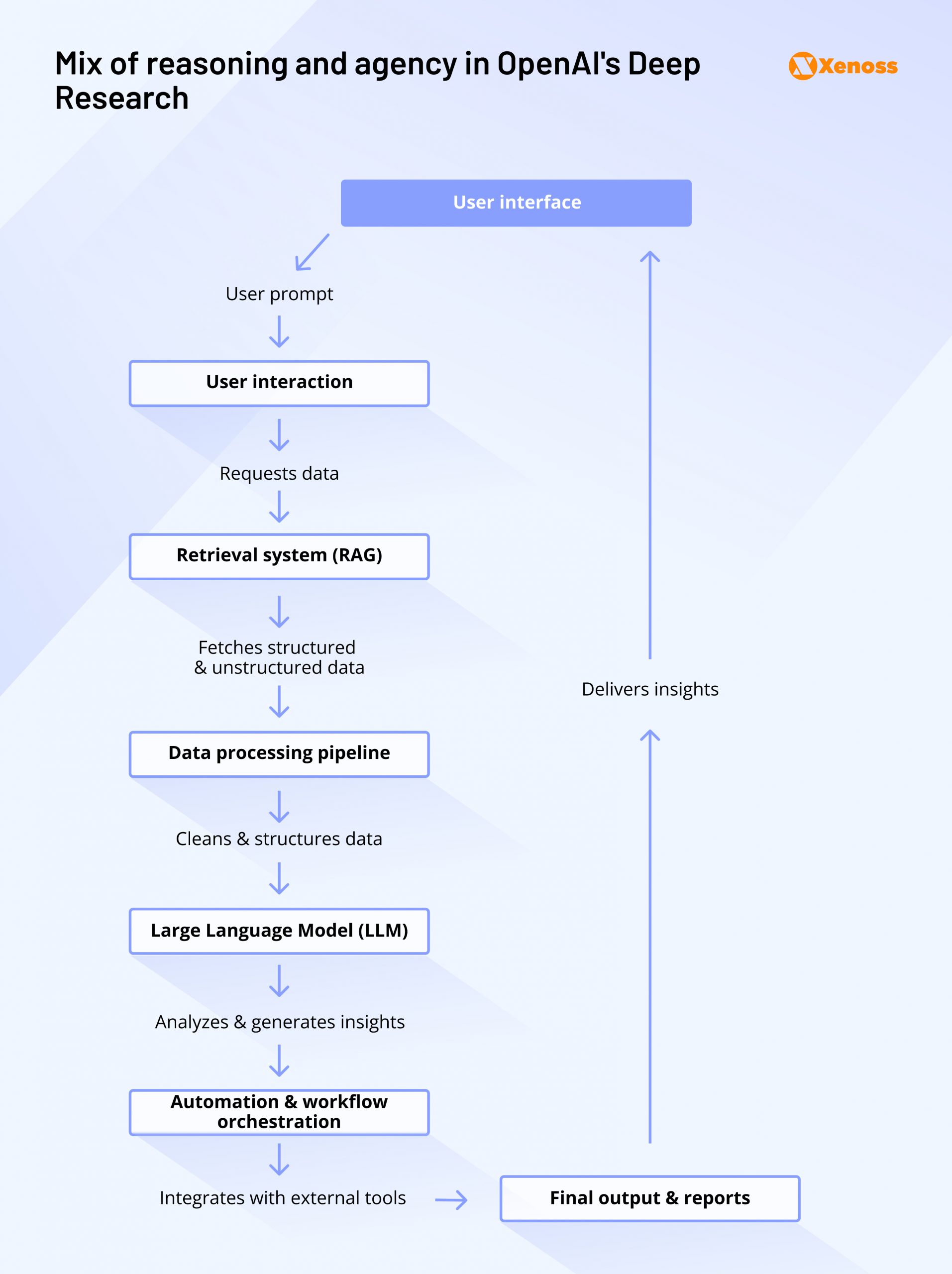
Practical example: Deep Research for bioinformatics market analysis
To gauge the potential of Deep Research in a real-world context, it was tasked to research and analyze the bioinformatics industry, specifically exploring how a data engineering and machine learning development company should navigate the sales cycle.
The prompt
To get a more detailed research output, a human supplied it with a detailed prompt.
Deep Research had to create a report featuring answers to questions across four thematic areas:
1. Pharma and Biotech companies
- Do pharma and biotech companies prefer building solutions in-house or outsourcing them? Back up your answer with evidence
- Why would companies avoid hiring outside developers? Back your answer with evidence.
- What types of in-house AI and data engineering solutions do pharma and biotech companies build?
- Show up-to-date examples. What type of engineers do biotech and pharma companies hire? What domain knowledge is required to be a successful AI/data engineering developer for a pharma company?
2. Trends in Bioinformatics
- What are the fastest-growing niches in bioinformatics?
- What recent innovations are shaping the sector?
3. Bioinformatics software companies
- How many bioinformatics software development companies are there?
- What types of projects are they building?
- Who are their clients?
4. Demand for AI and data engineers in biotech
- Is there demand for biotech/pharma software engineers?
- What barriers does a software company face when it comes to market entry?
- What criteria could facilitate the company’s entry into the market?
- What industry connections does a software engineering company need to enter the pharma/bioinformatics market?
- What kind of case studies and technologies would clients appreciate from a software development company planning on entering the pharma/biotech industry?
It also requested clarification on region, company size, and whether to include case studies or focus purely on trends. After clarifications, the agent started the research.
Research process
Deep Research openly shares its train of thought as it roams the web, allowing one to retrace the decision-making process and improve the trustworthiness of the final output.
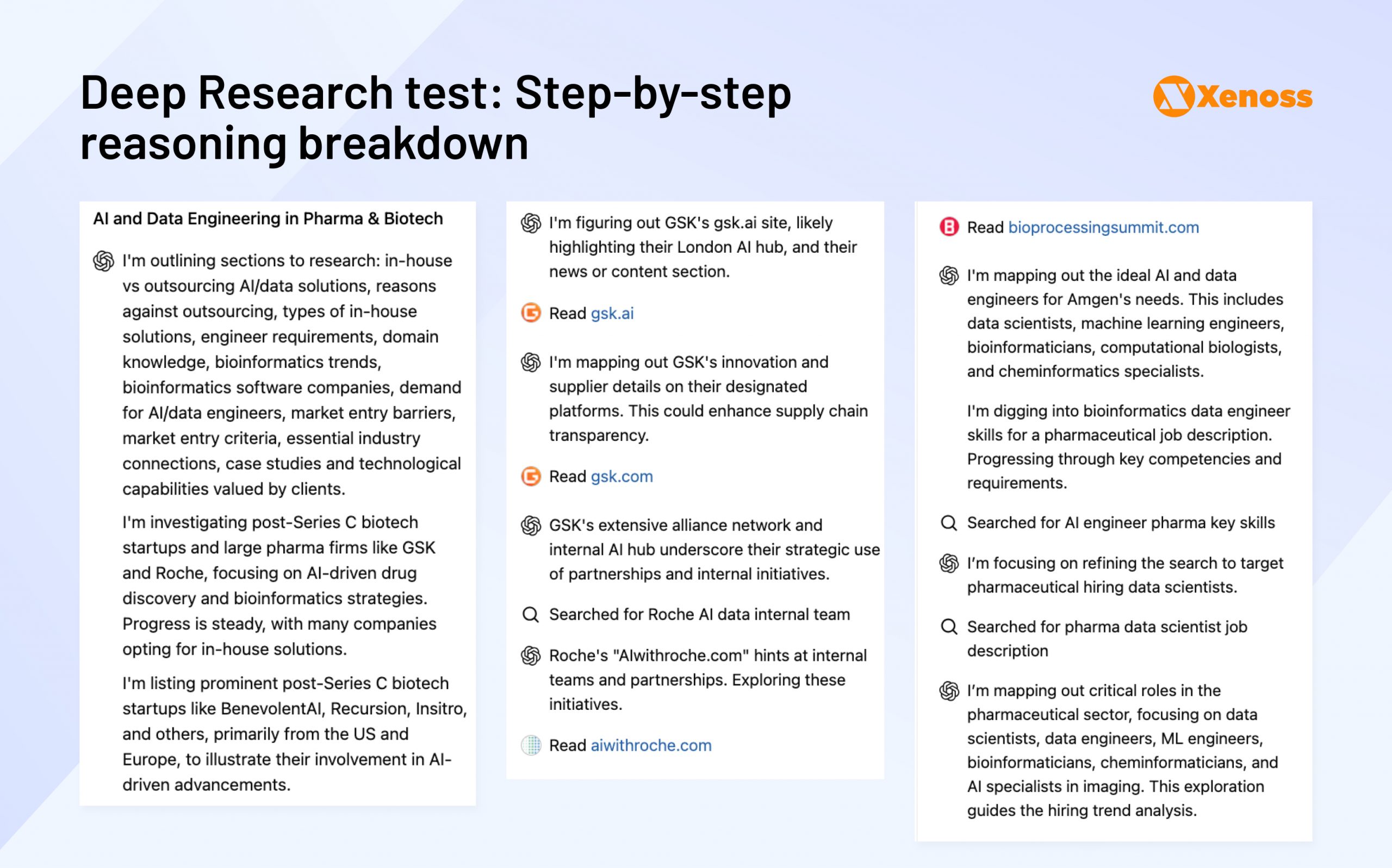
Notably, Deep Research even highlighted the technical errors it encountered along the way.
Importance of specifying research sources
In the first iteration of the research, the Xenoss team did not specify the sources that Deep Research should and should not use.
That is why, in the first output, the agent cited corporate blog posts that an in-house analyst typically considers unreliable.
To improve the credibility of the report, in the second iteration, the list of sources was limited to the following:
- Reports from industry leaders (e.g., McKinsey, BCG, IQVIA, Deloitte, CB Insights, Gartner, etc.).
- Social media posts from credible industry professionals (LinkedIn, Twitter/X, Medium posts from recognized experts, etc.).
- Business intelligence tools like Crunchbase for company data and investment trends.
- Regulatory body reports (FDA, EMA, NIH, etc.)
- Published research papers (PubMed, Nature Biotechnology, etc.)
- Insights from investor reports (VC firms, pharma investor briefings)
The tweak was successful. The second report on the same topic, now with source constraints, included more reliable citations.
It’s worth noting that, despite source restrictions, Deep Research occasionally reverted to less authoritative blog content, likely due to difficulty finding high-quality sources on niche market dynamics.
Where Deep Research did well
Operational gains
The most obvious (and substantial) benefit of Deep Research is its ability to produce a coherent and valuable report in under 10 minutes.
The depth of reasoning it demonstrated was, without being somewhat shallow, a seasoned bioinformatics expert would have blown GPT out of the park, but at what cost?
Producing a similar output manually would take human researchers days, pulling their focus away from tasks where an AI substitute isn’t yet viable.
Besides that, some human post-processing was still required. The original report was delivered in dense blocks of text, making it difficult to read without restructuring. Analysts had to spend time improving the flow and formatting for clarity.
Nevertheless, productivity gains were significant. Purpose-built agents like Deep Research can already bring measurable efficiency improvements, and leaders should consider integrating them into research workflows.
Human-like writing quality
While Deep Research currently runs on GPT o3, not GPT-4.5, recent improvements on the “personality of GPT-4o” make it feel more humanlike. This shift came through in the Deep Research test.
The pharma market findings were not significantly less readable than those written by a human researcher.
Human | Deep Research |
Overall, the bioinformatics market is expected to continue its robust growth trajectory, driven by increasing demand for personalized medicine, advancements in genomics and proteomics, and the integration of artificial intelligence and machine learning technologies in data analysis. Market size estimates are from 11 to 20 billion USD in 2023, with 13% growth predictions. There is a large number of open-source software and many SaaS and product companies. Initial research shows around 15 independent custom software development companies engaged in developing Bioinformatics solutions. | Large pharmaceutical companies increasingly favor in-house building AI and data capabilities, while smaller biotechs often turn to external partners. In clinical development, the trend has swung “back in” for big pharma – after years of heavy outsourcing to CROs, many larger firms are insourcing more R&D functions. These established players can afford to develop best-in-class AI/data teams internally and outsource only selectively. In contrast, emerging biotechs with fewer resources still rely heavily on outsourced expertise. Industry surveys show that the overall use of outsourcing in pharma R&D has risen – outsourced services grew from about one-third to nearly one-half of pharma’s spending from 2014 to 2023. |
Going beyond generic GPT outputs
Before the release of Deep Research, GPT struggled with answering questions with blanket statements that didn’t offer a unique view of the target field.
The Xenoss team wanted to examine how “deep” the analysis of Deep Research is and was reasonably satisfied with the output.
For example, it successfully highlighted the rise of health tech M&A by big pharma companies (a trend Xenoss market researchers have already spotted empirically).
Deep Research: “Yet many pharma companies also acquire AI startups to bring those capabilities in-house. In fact, big pharmas often bolster their “AI readiness” by buying innovative tech firms rather than depending solely on vendors.
We also see numerous partnerships: from 2022 to 2024, pharma-tech collaborations for AI rose ~30%, indicating companies often opt for external AI partnerships in the short term while strategizing to internalize critical capabilities long term.
In summary, pharma’s approach is hybrid, leveraging outside AI expertise for a quick boost but ultimately investing in in-house platforms (or acquisitions) to maintain control and IP. “
While Deep Research made an effort to cite data, it occasionally struggled to locate high-authority sources, particularly when addressing highly specific or emerging market dynamics.
All in all, though we do not believe Deep Research is capable of replacing consultants from top-tier firms yet, it does go a level deeper compared to the run-of-the-mill ChatGPT and can stand on par with a team of human researchers, assuming they don’t have access to insider knowledge.
Good for summarizing key points
Human and GPT market research yielded similar market summaries.
Human: “There are potential opportunities in the market; the market is growing and will continue to evolve. We have all the necessary IT skills. What we lack is medical knowledge. This problem can be solved by a partner organization, a guide.”
Deep Research: Pharma and biotech companies rapidly adopt AI and data engineering across drug discovery, clinical trials, bioinformatics, and manufacturing, creating strong demand for skilled engineers.
Despite significant barriers—including regulatory complexity, data privacy concerns, slow sales cycles, entrenched incumbents, and cautious organizational cultures—entry can be facilitated by demonstrating clear regulatory compliance, providing robust proof-of-value through real-world case studies, strategically partnering with established pharma companies and CROs, and leveraging influential industry connections and specialized niche solutions.
In both cases, partnering with domain-specific organizations was suggested as the ideal action.
Where Deep Research falls short
Tools like Deep Research are both an impressive technological leap and helpful assistants in day-to-day tasks. Still, at least the way things stand now, they are not a silver bullet to all business intelligence and research problems.
Here are a few shortcomings the team noticed in the output of Deep Research.
Source fidelity
As mentioned, not purging Deep Research from citing questionable corporate blogs was 100% effective. A quick Google Search confirmed that other Deep Research users face similar challenges.
Nuance detection
Our prompt clearly specified an interest in learning more about the software development outsourcing market.
A moderately sharp human researcher would have quickly realized how to tailor the report to the desired objective: Seeing what type of machine learning and data engineering services pharma companies bring on board and their vendor selection criteria.
However, Deep Research often cited examples related to manufacturing and supply chain partnerships—topics outside the intended scope.
Deep Research: “Finally, past experience with outsourcing mishaps (e.g, quality incidents at external manufacturers, which occurred at 91% of companies surveyed) makes pharma firms cautious. ”
Lack of insider insights
Occasionally, Deep Research would stumble upon good sources (like this excellent article on selling AI and data engineering services to pharma companies).
However, it paraphrased key insights into generic statements, missing the author’s hands-on experience working with enterprise pharma vendors—something human analysts would immediately recognize and emphasize.
Original piece:
There are three fundamental truths you need to understand if you want to use AI to create huge value in drug discovery:
- The further you are from the drug program decisions, the less value you create.
- The further the decisions you influence are from the commercial + clinical stages of drug discovery, the less value you create.
- The decisions that create the most value are choosing the right target/phenotype, reducing toxicity, predicting drug response, + choosing the right target population.
Deep Research (citing the article):
“Selling into big pharma can be a ‘black box’ to those not already in the industry. Large pharma companies have complex organizational structures and procurement processes.
A new software vendor might not know who the decision-makers are (IT, R&D, and procurement all have a say), and navigating this can take a long time. Moreover, big pharma typically has annual budget cycles and requires internal business sponsor buy-in at multiple levels. Compared to more agile industries, the sales cycle
in pharma for enterprise software can easily be 12–18 months from initial contact to a signed contract. ”
A lot more words, but a lot less substance.
Dated statistics
While Deep Research strives to use sources from 2024 and 2025, it does not have the human focus on tracking fresh news stories and contextualizing them.
For highly dynamic industries (as AI happens to be), not having up-to-date, context-aware data can significantly limit the report’s relevance and value.
Prompt limitations (Plus plan)
For Plus users, having access to only 10 prompts a month limits the ability to run conclusive pilot experiments. By limiting the testing grounds for Deep Research, OpenAI is tapering the tool’s impact.
That said, for enterprise teams investing in research acceleration, the $200/month plan is a relatively modest cost, especially when weighed against the time saved.
It would be interesting to see how Deep Research transforms the quality of research and analytical tasks once it becomes more accessible to lower subscription tiers (which might happen quite soon, considering Google’s Deep Research, powered by Gemini, is now free).
Best practices for prompting Deep Research
To ensure the model does not stray from the desired direction, make it clear with point-based questions and well-defined constraints, as if you are giving a task to a human.
By trial and error, the Xenoss team derived the following best practices:
- Use other GPT models to flesh out your prompts. Feeding the standard GPT interface a list of starter questions and objectives was helpful. GPT would expand upon target areas of interest, creating a more nuanced prompt.
- Regulate the verbosity of research. Like standard GPT models, DeepSearch offers highly detailed answers, where the same ideas are often phrased differently. Consider adding style instructions (e.g., “Be concise”) to prompts.
- Guide Deep Research to back up statements with evidence (statistics or case studies).
As for the structure, here’s the “Prompt anatomy” framework used to improve the output with each subsequent iteration.
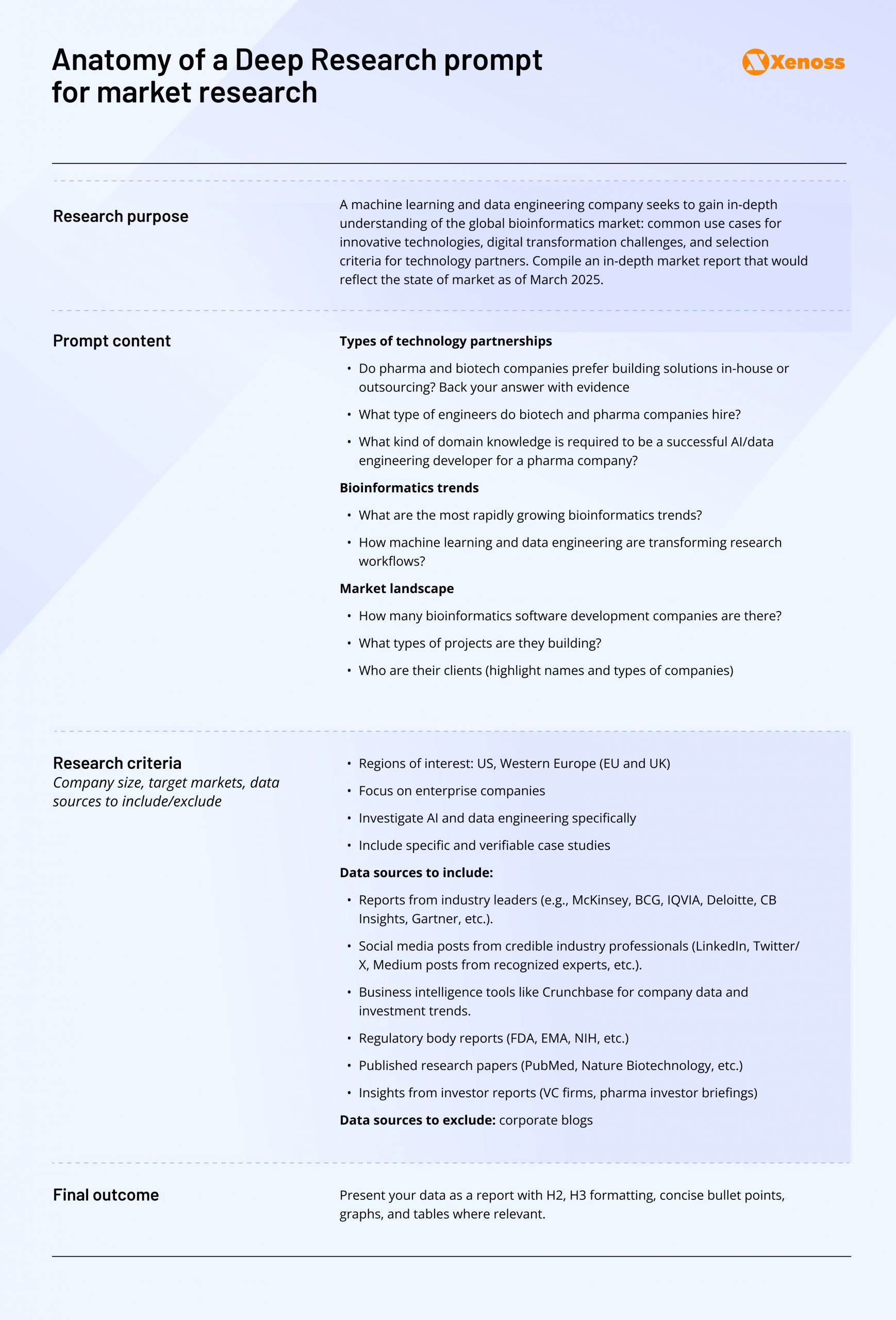
Bottom line
OpenAI’s Deep Research isn’t ready for high-stakes strategic decisions (global expansion or launching new products). The Xenoss team’s experiments showed that the current Deep Research is still a tool rather than a fully “thinking entity”. It is powerful but not without extensive fact-checking.
As for promising use cases, the Xenoss team found it excellent to offer a helicopter view of a target market that can drive the course of future investigations.
GPT’s ever-evolving writing will soon make Deep Research a content team’s go-to assistant for writing blog posts and white papers.
The value of Deep Research lies in augmentation, not automation. A new generation of purpose-built AI agents can quickly get analysts and consultants started on preliminary market research that will be reviewed and expanded upon by humans with insider knowledge of target industries.